Artificial Intelligence (AI) has become an integral part of our daily lives, transforming the way we interact with technology and the world around us. From virtual assistants to personalized recommendations, AI is reshaping various aspects of our existence. Let’s explore 50 points highlighting the profound impact of AI on everyday life:
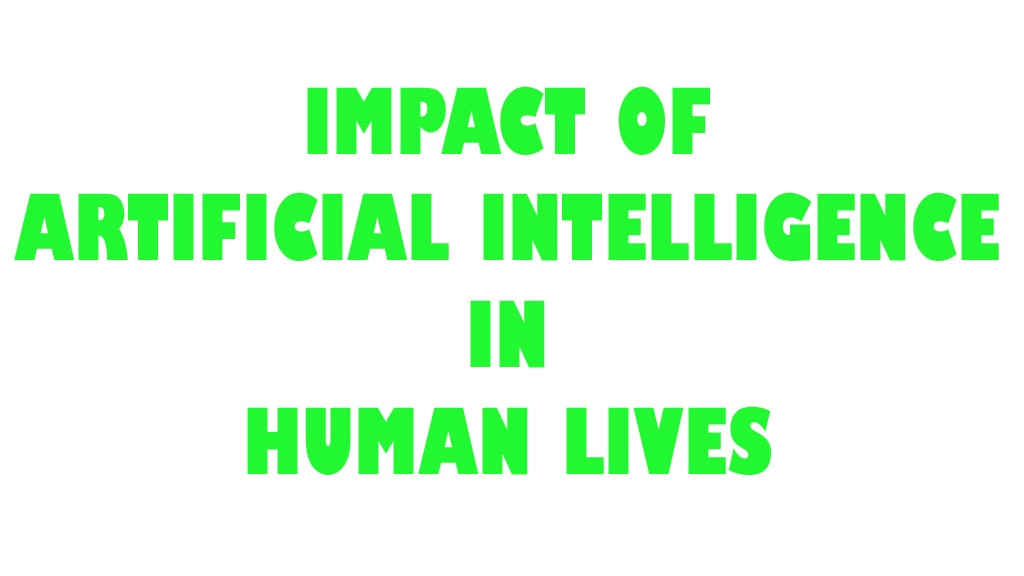
#1 AI in communication and information
AI plays a significant role in communication and information management, revolutionizing how we interact with technology and access information. Here are some key ways AI is integrated into communication and information systems:
1. **Natural Language Processing (NLP)**: NLP is a core component of AI that enables computers to understand, interpret, and generate human language. AI-powered chatbots and virtual assistants use NLP to understand user queries and provide relevant responses, whether it’s answering questions, offering customer support, or engaging in casual conversations.
2. **Information Retrieval**: AI algorithms are used to enhance search engines, making them more efficient at finding relevant information. Search engines like Google use AI techniques to understand search intent, context, and user behavior to deliver more accurate and personalized search results.
3. **Language Translation**: AI-driven language translation services have improved significantly in recent years. They utilize deep learning techniques, such as neural machine translation, to translate text and speech between different languages, facilitating global communication.
4. **Sentiment Analysis**: AI can analyze and interpret the sentiment behind textual data, such as social media posts, reviews, and customer feedback. This helps organizations understand public opinion and sentiment towards their products, services, or events.
5. **Speech Recognition**: AI-based speech recognition systems convert spoken language into text, enabling hands-free interaction with devices and applications. They power virtual assistants and voice-controlled interfaces in various devices.
6. **Personalization**: AI algorithms collect and analyze user data to personalize communication and information delivery. Whether it’s recommending products, suggesting content, or tailoring marketing messages, AI helps create more personalized experiences for users.
7. **Content Creation**: AI can generate human-like text and content through natural language generation techniques. This has applications in content creation for news articles, product descriptions, personalized marketing messages, and more.
8. **Predictive Text Input**: AI-based keyboards and text input systems use predictive text suggestions based on the context of the conversation, making typing faster and more efficient.
9. **Information Security**: AI helps enhance information security by identifying potential threats and anomalies in network traffic, detecting phishing attempts, and safeguarding sensitive data.
10. **Social Media Management**: AI tools assist in managing social media accounts by scheduling posts, analyzing engagement metrics, and suggesting content to improve social media presence.
11. **Data Analysis**: AI algorithms can process vast amounts of data to uncover patterns, insights, and trends, helping businesses and researchers make data-driven decisions.
12. **Automated Customer Service**: AI-driven chatbots and virtual assistants handle customer queries and provide support 24/7, improving customer service efficiency and reducing response times.
Overall, AI’s integration in communication and information management has led to more efficient, personalized, and interactive experiences for users across various platforms and industries.
#2 AI enabling hands-free control of our devices, enhancing convenience.
AI can enable hands-free control of devices and enhance convenience through various methods and technologies. Here are some key ways AI can achieve this:
1. **Voice Recognition:** AI-powered voice recognition systems like Siri, Google Assistant, or Alexa allow users to interact with their devices using natural language commands. This enables hands-free control of various tasks like setting alarms, sending messages, making calls, playing music, getting directions, and more.
2. **Gesture Recognition:** AI can interpret hand gestures and movements through cameras or sensors on devices like smartphones, tablets, or gaming consoles. This allows users to control their devices without touching the screen, making it convenient in situations where hands are occupied or messy.
3. **Facial Recognition:** AI-powered facial recognition technology can identify users and personalize their experience on devices without requiring any physical input like passwords or PINs. This can enable hands-free unlocking of smartphones or laptops.
4. **Contextual Awareness:** AI can analyze user behavior, preferences, and environmental data to provide personalized and contextually relevant information. For instance, a device could automatically adjust its settings based on a user’s location, time of day, or previous interactions, reducing the need for manual input.
5. **Predictive Assistants:** AI assistants can learn from user behavior and anticipate their needs, proactively providing information or suggestions without direct prompting. This can save time and effort by automating routine tasks.
6. **Home Automation:** AI-powered smart home devices can be controlled using voice commands, allowing users to manage lighting, temperature, security systems, and appliances without physical interactions.
7. **Accessibility Features:** AI can enhance the accessibility of devices for people with disabilities. For example, speech recognition and text-to-speech technologies can help individuals with limited mobility control their devices using their voice.
8. **Autonomous Vehicles:** AI is crucial in enabling hands-free driving in autonomous vehicles. The AI algorithms control the vehicle’s movements, making it possible for passengers to focus on other tasks during the ride.
9. **Virtual and Augmented Reality:** AI-powered virtual and augmented reality systems can interpret natural language and gestures, enabling users to interact with virtual environments and digital content without using physical controls.
10. **Healthcare Applications:** AI can enable hands-free control of medical devices and healthcare systems, benefiting patients and medical professionals alike. For example, surgeons can use voice commands to access patient data during operations, and patients can interact with medical devices using gestures or voice commands.
By leveraging AI’s capabilities, devices become more intuitive and responsive, reducing the need for manual inputs and making the user experience more convenient and seamless. However, it is essential to balance convenience with privacy and security considerations to ensure that AI-powered devices respect users’ boundaries and protect their data.
#3 AI algorithms analyze data to provide personalized recommendations on streaming platforms
AI algorithms analyze data to provide personalized recommendations on streaming platforms using a combination of data processing, machine learning, and user behavior analysis. Here’s a high-level overview of how this process typically works:
1. Data Collection: Streaming platforms gather various types of data from their users. This data includes user interactions, such as watching history, search queries, ratings, likes, and dislikes, as well as demographic information like age, gender, and location. Additionally, they may collect data on the content itself, including metadata, genre, actors, directors, and other relevant information.
2. Data Preprocessing: The collected data goes through a preprocessing phase to clean and organize it. This step may involve removing duplicates, handling missing values, and transforming data into a suitable format for analysis.
3. Feature Extraction: Features or attributes are derived from the preprocessed data to represent different aspects of user behavior and content characteristics. These features can include user preferences, content genres, popularity, release date, and more.
4. Creating User Profiles: AI algorithms build user profiles by analyzing historical user interactions and behaviors. These profiles capture individual preferences, viewing habits, and interests. The goal is to understand what content a user may enjoy and engage with the most.
5. Content Analysis: Similarly, the AI algorithms analyze the content itself to extract relevant features and create content profiles. This helps in understanding the characteristics and genre of each piece of content.
6. Machine Learning Models: The heart of personalized recommendations lies in machine learning models. The platform uses various recommendation algorithms, such as collaborative filtering, content-based filtering, matrix factorization, and deep learning models, to process the user and content profiles. These models learn from historical data to make predictions about a user’s preferences and interests.
7. Recommendation Generation: Based on the machine learning models’ predictions, the system generates a list of recommended content for each user. The recommendations may include movies, TV shows, music, or other types of content available on the platform.
8. Real-Time Personalization: Streaming platforms often employ real-time personalization to adapt recommendations on the fly as users interact with the platform. User actions, such as clicks, watches, and ratings, continuously update the user profiles and, consequently, the recommendations.
9. A/B Testing: To continually improve the recommendation system’s effectiveness, streaming platforms often perform A/B testing. They compare different recommendation algorithms and fine-tune the models based on user feedback and engagement metrics.
10. User Feedback Loop: User feedback, such as explicit ratings or feedback on recommended content, is essential to refine the recommendations further. This feedback is incorporated back into the system to improve the accuracy of future recommendations.
Overall, the process of personalized recommendations on streaming platforms is a continuous cycle of data collection, analysis, and model refinement. The goal is to provide users with the most relevant and engaging content, enhancing their overall experience on the platform.
#4 AI chatbots improve customer support and engagement
AI chatbots can indeed significantly improve customer support and engagement in various ways. Here are some of the key benefits of using AI chatbots in customer service:
1. 24/7 Availability: AI chatbots can operate around the clock, providing instant support to customers at any time of day or night. This ensures that customers’ inquiries and issues are addressed promptly, regardless of the time zone or business hours.
2. Swift Responses: AI chatbots can process and analyze large amounts of data in real-time, allowing them to provide quick and accurate responses to customers’ queries. This efficiency saves customers’ time and enhances their overall experience.
3. Scalability: Unlike human agents, AI chatbots can handle multiple customer interactions simultaneously without any drop in the quality of service. This makes them highly scalable and cost-effective for businesses dealing with large volumes of customer inquiries.
4. Reduced Waiting Times: AI chatbots can handle routine and straightforward queries, freeing up human agents to focus on more complex issues. This reduces the waiting time for customers, leading to higher satisfaction levels.
5. Personalization: Advanced AI chatbots can analyze customer data and past interactions to personalize their responses. By understanding customer preferences and history, chatbots can offer tailored recommendations and solutions, making customers feel valued.
6. Consistency: AI chatbots provide consistent responses and adhere to predefined rules and guidelines set by the business. This ensures that customers receive uniform information, minimizing the risk of misinformation.
7. Multilingual Support: AI chatbots can be programmed to support multiple languages, making them suitable for businesses with a global customer base. This eliminates language barriers and improves accessibility for international customers.
8. Data Collection and Analysis: AI chatbots can gather valuable customer data and feedback during interactions. This information can be analyzed to identify patterns, preferences, and pain points, enabling businesses to make data-driven improvements to their products and services.
9. Cost Savings: By automating routine customer support tasks, businesses can save on operational costs associated with hiring and training additional human agents.
10. Continuous Improvement: AI chatbots can learn from each interaction and improve their performance over time. With machine learning algorithms, they can become more intelligent and accurate in addressing customer needs.
However, it’s important to note that while AI chatbots are highly beneficial for customer support, they may not replace human agents entirely. Some complex issues or situations may still require the empathy and problem-solving abilities that only human representatives can provide. Therefore, a balanced approach that combines the strengths of both AI chatbots and human agents is often the most effective strategy for enhancing customer support and engagement.
#5 AI enhances healthcare
AI has the potential to revolutionize healthcare in numerous ways, providing significant benefits for both patients and healthcare providers. Some of the key ways AI enhances healthcare include:
1. **Medical Imaging and Diagnostics:** AI can analyze medical images, such as X-rays, CT scans, and MRIs, with great precision and speed. It can help identify patterns and anomalies that might be difficult for human eyes to detect, leading to more accurate and early diagnoses of diseases like cancer, neurological disorders, and more.
2. **Personalized Treatment Plans:** AI can analyze vast amounts of patient data, including medical records, genetic information, and lifestyle factors, to tailor treatment plans for individual patients. This approach improves treatment effectiveness and reduces the risk of adverse reactions to medications.
3. **Drug Discovery and Development:** AI can significantly accelerate the drug discovery process by analyzing molecular interactions, predicting potential drug candidates, and simulating their effects on the human body. This expedites the identification of new drugs and therapies.
4. **Health Monitoring and Predictive Analytics:** AI-powered wearables and remote monitoring devices can continuously track patients’ vital signs and health parameters. By analyzing this data, AI can detect early warning signs of health issues and predict potential health risks, enabling timely interventions.
5. **Virtual Health Assistants and Chatbots:** AI-driven virtual health assistants can provide patients with immediate access to medical information, answer questions, and offer basic medical advice. They can also help schedule appointments and remind patients to take their medications.
6. **Administrative Efficiency:** AI can streamline administrative tasks, such as billing and coding, scheduling, and data entry, allowing healthcare providers to focus more on patient care and reducing the administrative burden.
7. **Natural Language Processing for Medical Documentation:** AI-powered natural language processing (NLP) systems can transcribe and analyze medical notes, making it easier for healthcare professionals to access and update patient records accurately and efficiently.
8. **Healthcare Fraud Detection:** AI algorithms can analyze claims data to identify patterns of fraudulent or abusive behavior, helping to prevent healthcare fraud and reduce overall healthcare costs.
9. **Robot-Assisted Surgery:** AI-enabled surgical robots can enhance surgical precision and reduce invasiveness, leading to faster recovery times and improved patient outcomes.
10. **Epidemiological Surveillance:** AI can analyze vast amounts of data from various sources, such as social media, news reports, and health records, to detect disease outbreaks and monitor the spread of infectious diseases in real-time.
11. **Mental Health Support:** AI-powered mental health apps and chatbots can provide personalized support and coping mechanisms for individuals experiencing stress, anxiety, or depression.
However, it’s important to note that while AI offers promising advancements, it should always complement and enhance human expertise rather than replace it. Ethical considerations, data privacy, and maintaining human oversight are crucial aspects that need careful attention when implementing AI in healthcare.
#6 AI-powered wearable devices track health metrics, encouraging healthier lifestyle choices
AI-powered wearable devices can track health metrics and encourage healthier lifestyle choices through a combination of advanced sensors, data analysis, and personalized feedback. Here’s how they work:
1. **Sensor Technology:** Wearable devices are equipped with various sensors like heart rate monitors, accelerometers, gyroscopes, GPS, temperature sensors, and more. These sensors continuously collect data about the wearer’s body and its surroundings.
2. **Data Collection:** The collected data includes vital signs like heart rate, blood pressure, respiratory rate, sleep patterns, activity levels, and even environmental factors like air quality and temperature. Some wearables can also monitor glucose levels for diabetics and analyze sweat to detect dehydration or other health markers.
3. **Data Analysis with AI:** The raw data collected by wearables is processed and analyzed using AI algorithms. These algorithms can identify patterns, trends, and anomalies in the data to gain insights into the wearer’s health and behavior.
4. **Health Metrics and Insights:** AI algorithms can interpret the data to generate health metrics and insights about the wearer’s overall health status. For example, it can provide information about the number of steps taken, calories burned, sleep quality, stress levels, and more.
5. **Personalized Recommendations:** Based on the analysis of the collected data, AI algorithms can provide personalized recommendations and suggestions to encourage healthier lifestyle choices. For instance, if the device detects insufficient physical activity, it might prompt the user to take a short walk or suggest suitable exercises. If the user’s sleep patterns are irregular, it could offer tips for improving sleep hygiene.
6. **Goal Setting and Gamification:** Wearable devices often allow users to set health and fitness goals. AI can help in setting realistic and achievable targets based on the user’s current health status. To motivate users, wearables often employ gamification elements, such as rewards, badges, or friendly competitions with friends or other users.
7. **Real-Time Feedback:** Wearable devices can provide real-time feedback to users, helping them stay on track with their health goals. For instance, it can remind them to stand up and move if they have been sitting for too long, or it can offer breathing exercises to reduce stress levels.
8. **Data Sharing and Insights:** Many wearable devices are connected to smartphones or other smart devices. Users can share their health data with healthcare professionals, fitness trainers, or family members to receive additional guidance and support.
9. **Continuous Learning and Improvement:** AI algorithms in wearable devices can continuously learn from user data, refining their recommendations over time to offer more accurate and personalized guidance.
By combining these technologies and features, AI-powered wearable devices can play a significant role in tracking health metrics and motivating individuals to make healthier lifestyle choices. They empower users to be more aware of their health and take proactive steps to improve their overall well-being.
#7 AI enables personalized learning experiences, adapting educational content to individual student needs
AI enables personalized learning experiences by leveraging advanced algorithms and data analysis techniques to tailor educational content to individual student needs. Here’s how it works:
1. **Data Collection and Analysis**: AI systems gather vast amounts of data on students’ learning behaviors, preferences, strengths, weaknesses, and historical performance. This data can include various sources, such as student interactions with educational software, assessment results, teacher feedback, and even external factors like demographics and learning environment.
2. **Student Profiling**: Using the collected data, AI creates individualized profiles for each student. These profiles are dynamic and continuously updated as the AI learns more about the student’s progress and learning patterns.
3. **Adaptive Content Selection**: Based on the student profiles, the AI algorithm selects and recommends appropriate educational content that matches each student’s learning style, pace, and preferences. For example, if a student is struggling with a specific concept, the AI might recommend additional resources or practice exercises to reinforce understanding.
4. **Learning Pathways**: AI can create customized learning pathways for each student, guiding them through a sequence of educational materials and activities that suit their unique needs. Advanced algorithms predict the most effective sequence of topics and concepts for optimal learning outcomes.
5. **Real-Time Feedback and Assessment**: AI can provide immediate feedback to students on their performance, allowing them to address mistakes and misconceptions promptly. Additionally, AI systems can continuously assess the student’s progress and adjust the content accordingly to keep the learning experience challenging yet achievable.
6. **Natural Language Processing (NLP)**: NLP allows AI to interact with students using natural language, making the learning process feel more interactive and engaging. Students can ask questions, seek explanations, or engage in discussions with the AI-powered educational tools.
7. **Personalized Recommendations**: AI can recommend additional learning materials, courses, or activities based on a student’s interests and goals, encouraging self-directed learning and exploration.
8. **Identifying Learning Difficulties**: AI can help educators identify potential learning difficulties or learning disabilities in students by analyzing their interactions and performance. Early detection allows for timely interventions and support.
9. **Teacher Support**: AI-powered tools can assist teachers by providing insights into each student’s progress, suggesting personalized interventions, and automating administrative tasks, allowing educators to focus more on individualized instruction and mentorship.
Overall, AI enables personalized learning experiences by leveraging technology to cater to each student’s specific needs, preferences, and abilities, enhancing the effectiveness and efficiency of the education process. However, it’s essential to balance the benefits of AI with concerns about data privacy, algorithm bias, and the need for human interaction and guidance in the learning process.
#8 Autonomous vehicles, driven by AI
Autonomous vehicles, also known as self-driving cars, are vehicles that can operate and navigate without direct human intervention. These vehicles rely on a combination of artificial intelligence (AI), sensors, cameras, and other advanced technologies to perceive their surroundings, make decisions, and control their movements. The development of autonomous vehicles involves several key components and processes:
1. Sensing Technology: Autonomous vehicles are equipped with a variety of sensors, including radar, lidar, cameras, ultrasonic sensors, and GPS. These sensors work together to collect data about the vehicle’s surroundings, such as the position of other vehicles, pedestrians, road markings, and traffic signals.
2. Perception and Mapping: The collected sensor data is processed by AI algorithms to create a detailed and real-time map of the vehicle’s environment. The AI system must accurately recognize and interpret objects, predict their movements, and understand the overall traffic situation.
3. Decision-Making: AI algorithms in the vehicle’s onboard computer analyze the sensor data and map information to make real-time decisions. These decisions involve determining the appropriate speed, acceleration, braking, and lane changes to navigate safely and efficiently.
4. Control and Actuation: Based on the decisions made by the AI, the vehicle’s control system takes action to manipulate the steering, throttle, and brakes to execute the planned maneuvers.
5. Machine Learning: To improve performance and adapt to various scenarios, autonomous vehicles rely heavily on machine learning. They continually learn from the vast amounts of data generated during their operations and use this knowledge to enhance their driving capabilities.
6. Connectivity: Autonomous vehicles often rely on communication technology to exchange data with other vehicles and infrastructure elements, such as traffic lights and road signs. This connectivity can further enhance safety and efficiency.
7. Safety and Redundancy: Safety is a paramount concern in autonomous vehicle development. Manufacturers implement redundant systems and fail-safe mechanisms to ensure that the vehicle can handle unexpected situations or malfunctions.
8. Testing and Validation: Autonomous vehicles undergo extensive testing, both in controlled environments and on public roads. This testing helps identify and address potential issues, refine the AI algorithms, and build public trust in the technology’s safety.
9. Legal and Regulatory Framework: The deployment of autonomous vehicles involves navigating through complex legal and regulatory challenges. Governments need to establish guidelines and standards to ensure the safe and responsible use of these vehicles on public roads.
10. Gradual Deployment: The integration of autonomous vehicles into our transportation systems is expected to be a gradual process. Initially, they may operate in controlled environments, such as designated autonomous lanes or closed areas, and then slowly expand to broader usage as their capabilities and safety are proven.
It’s important to note that the field of autonomous vehicles is continuously evolving, and ongoing research and development are essential to address challenges and improve the technology further. As of my last update in September 2021, autonomous vehicles were already being tested on public roads, but widespread adoption was still in progress, subject to technological advancements, regulatory approval, and public acceptance.
#9 AI optimizes route planning, reducing travel times and improving traffic management
AI optimizes route planning and improves traffic management through various techniques and technologies. Here are some of the key ways AI accomplishes this:
1. Data Analysis and Prediction: AI can process large volumes of data from various sources, such as GPS devices, traffic cameras, historical traffic patterns, and real-time updates from mobile apps. By analyzing this data, AI can predict traffic congestion, identify high-traffic areas, and anticipate potential bottlenecks, enabling it to suggest alternative routes to avoid delays.
2. Real-Time Updates: AI can provide real-time traffic updates to drivers based on current road conditions. By continuously monitoring traffic flow and incidents, it can suggest dynamic route adjustments to avoid accidents, construction zones, or other unexpected events.
3. Machine Learning Algorithms: AI uses machine learning algorithms to learn from historical data and driver behavior. These algorithms can improve route recommendations over time by recognizing patterns, finding the most efficient routes, and adapting to changing traffic conditions.
4. Traffic Signal Optimization: AI can optimize traffic signal timings at intersections based on real-time traffic flow. By adjusting signal timings dynamically, it helps in reducing congestion and ensuring smoother traffic movement.
5. Adaptive Navigation Systems: AI-powered navigation systems can adapt to individual driving patterns and preferences. They can take into account factors like preferred routes, time of day, and historical travel patterns to provide personalized and efficient route suggestions.
6. Multi-Modal Transportation Planning: AI can integrate information from various modes of transportation, including public transit, rideshares, and cycling options. By offering multi-modal route suggestions, it encourages the use of alternative transportation methods, reducing overall traffic congestion.
7. Incident Management: AI can assist traffic management authorities in handling incidents promptly. By analyzing data from various sources, it can quickly identify accidents, breakdowns, or road closures, enabling traffic controllers to respond more efficiently.
8. Simulation and Modeling: AI can create traffic simulations and models that help urban planners and traffic engineers predict the impact of new infrastructure projects or changes to existing road networks. This allows for better decision-making to alleviate potential traffic issues.
9. Demand Prediction: AI can predict the demand for transportation services based on historical data, events, or even weather conditions. By anticipating spikes in travel demand, transportation providers can better prepare and allocate resources accordingly.
By leveraging AI’s capabilities in data analysis, prediction, and real-time decision-making, route planning and traffic management can be significantly improved. This not only reduces travel times for individual drivers but also leads to more efficient and sustainable transportation systems for cities and regions as a whole.
#10 AI helps detect fraudulent activities
AI can play a crucial role in detecting fraudulent activities across various industries and domains. The application of AI in fraud detection is based on its ability to analyze large volumes of data quickly and identify patterns that may be indicative of fraudulent behavior. Here’s how AI helps detect fraudulent activities:
1. Anomaly detection: AI systems can be trained on historical data to understand what “normal” behavior looks like within a system or process. By doing so, the AI can detect anomalies or deviations from the norm, which could indicate potential fraud. For example, if an individual’s spending patterns suddenly change drastically or if a transaction is significantly different from usual, it might be flagged as suspicious by the AI system.
2. Pattern recognition: AI can recognize patterns of fraudulent behavior that may be challenging for humans to spot due to the vast amount of data involved. It can identify similarities between known fraud cases and ongoing transactions, helping to identify potential fraud in real-time.
3. Machine learning algorithms: AI systems can utilize various machine learning algorithms to continuously improve their fraud detection capabilities. These algorithms can learn from new data and adapt to evolving fraud techniques, making the detection process more accurate and efficient over time.
4. Natural Language Processing (NLP): NLP is a branch of AI that allows machines to understand and interpret human language. It can be used to analyze unstructured data, such as text from customer reviews, social media, or support tickets, to identify any fraudulent activities or intentions.
5. Network analysis: In cases of fraud involving networks, AI can analyze relationships and connections among different entities, detecting complex fraud schemes involving multiple parties.
6. Real-time monitoring: AI-powered systems can monitor transactions and activities in real-time, instantly flagging suspicious behavior for further investigation. This proactive approach helps prevent fraudulent transactions from being successful.
7. Behavioral biometrics: AI can analyze unique behavioral patterns of users, such as typing speed, mouse movements, and touchscreen interactions. By monitoring these patterns, AI can detect if someone other than the legitimate user is attempting to access an account or perform a transaction.
8. Predictive analytics: AI can predict potential fraudulent activities based on historical data and trends. This enables businesses to take preventive measures before fraud occurs.
9. Integration with existing systems: AI fraud detection tools can be seamlessly integrated into existing software and processes, allowing organizations to enhance their security measures without significant disruption.
Overall, AI’s ability to process vast amounts of data, learn from it, and identify intricate patterns makes it a powerful tool in the fight against fraudulent activities, helping businesses and organizations stay one step ahead of fraudsters.
#11 AI automates repetitive tasks in workplaces
AI automates repetitive tasks in workplaces through a combination of advanced technologies and algorithms. Here’s how it typically works:
1. Data Collection: AI systems require data to learn and understand patterns. For automation, relevant data related to the repetitive task is collected and organized. This data can be structured (e.g., spreadsheets, databases) or unstructured (e.g., text, images).
2. Machine Learning: Machine learning algorithms analyze the collected data to identify patterns, correlations, and trends. Supervised learning, unsupervised learning, or reinforcement learning techniques may be used, depending on the task at hand.
3. Model Training: Based on the insights gained from the data, AI models are trained to perform the task accurately. During the training process, the model learns to generalize from the data, enabling it to handle variations and new instances of the repetitive task.
4. Decision Making: Once the AI model is trained, it becomes capable of making decisions and predictions related to the task. This decision-making ability is often based on probabilities and confidence levels derived from the training data.
5. Integration with Workflows: The trained AI model is integrated into the workplace’s existing systems and workflows. It can be deployed on servers, in the cloud, or even on edge devices, depending on the requirements and accessibility.
6. Task Automation: With the AI model integrated, it can now automatically perform the repetitive task based on the input it receives. This can range from simple rule-based tasks to more complex tasks that involve natural language processing, computer vision, or other specialized AI capabilities.
7. Continuous Improvement: AI systems can be designed to continuously learn and improve their performance over time. They may utilize techniques like online learning, reinforcement learning, or periodic retraining to adapt to changing data patterns and improve their accuracy.
Examples of AI automating repetitive tasks:
a. Data Entry: AI can process and extract information from documents, such as invoices, forms, and receipts, and populate relevant data into databases automatically.
b. Customer Support: AI-powered chatbots can handle routine customer queries and support requests, freeing up human agents to focus on more complex issues.
c. Email Filtering: AI algorithms can categorize and prioritize incoming emails, identifying spam and sorting messages into appropriate folders.
d. Quality Control: AI-based vision systems can inspect products on production lines for defects and inconsistencies, ensuring consistent quality.
e. Financial Analysis: AI can automate data analysis, generating reports, and identifying trends in financial data.
f. Language Translation: AI can automatically translate text or speech from one language to another, enabling multilingual communication.
g. Scheduling and Calendar Management: AI assistants can help manage schedules, book appointments, and set reminders.
By automating repetitive tasks, AI can increase efficiency, reduce errors, and save valuable time and resources for businesses, ultimately leading to improved productivity and competitiveness.
#12 AI improves language translation
AI improves language translation through the application of advanced algorithms and machine learning techniques. The primary approach used for language translation with AI is called Neural Machine Translation (NMT), which has shown significant improvements over traditional methods like rule-based or statistical machine translation.
Here’s how AI, particularly NMT, enhances language translation:
1. Neural Networks: NMT utilizes neural networks, specifically deep learning models like Recurrent Neural Networks (RNNs) and Transformer-based models, such as the popular “Transformer” architecture. These neural networks can learn complex patterns and dependencies in language data, enabling them to better capture the meaning of sentences and phrases.
2. Training on Large Datasets: AI models require vast amounts of data to learn effectively. NMT models are trained on extensive bilingual datasets containing millions of sentence pairs in different languages. The neural networks learn to map input sentences from one language to corresponding sentences in another language.
3. Contextual Understanding: Neural networks in NMT have the ability to grasp the context of a sentence, allowing them to generate more accurate translations. By considering the surrounding words and phrases, the model can make better decisions about the most appropriate translation for a given input.
4. Transfer Learning: Pretrained language models, such as BERT (Bidirectional Encoder Representations from Transformers), can be fine-tuned for translation tasks. These models have already learned a great deal about language and can be adapted to translation-specific tasks with additional training.
5. Attention Mechanism: The Transformer architecture introduced the attention mechanism, which helps the model focus on relevant parts of the input sentence during translation. This allows the model to pay more attention to critical words and phrases that contribute to the overall meaning of the sentence.
6. Continuous Learning: AI models can be continually updated and improved. When new data becomes available, the model can be retrained, allowing it to adapt to changes in language usage and maintain up-to-date translation capabilities.
7. Reduced Human Intervention: Compared to rule-based translation systems that require extensive manual crafting of rules, NMT requires less human intervention. The model learns directly from data, reducing the need for labor-intensive manual work and making it easier to scale to multiple language pairs.
8. Customization for Specific Domains: NMT models can be fine-tuned or specialized for specific domains, like legal, medical, or technical translations. This customization improves accuracy in those specific areas, ensuring more relevant and precise translations for domain-specific content.
Overall, AI-powered language translation benefits from the ability of neural networks to learn from vast amounts of data, understand context, and continuously improve, resulting in more natural and accurate translations across various languages.
#13 AI-powered robots assist in dangerous tasks
AI-powered robots assist in dangerous tasks by leveraging their advanced capabilities in perception, decision-making, and physical execution to perform activities that would be hazardous for humans. Here are some ways in which AI-powered robots are used to handle dangerous tasks:
1. Disaster Response: AI-powered robots can be deployed in disaster-stricken areas to search for survivors in collapsed buildings or hazardous environments, where human rescuers might be at risk. These robots are equipped with sensors and cameras to detect and locate people in need of help.
2. Bomb Disposal: Robots are frequently used to disarm explosive devices and handle potentially dangerous situations involving bombs or other hazardous materials. They can be remotely controlled by trained experts who ensure safe and precise operations.
3. Nuclear Plant Maintenance: In nuclear power plants, AI-powered robots can perform maintenance tasks in radioactive areas, reducing the exposure of human workers to harmful radiation.
4. Mining and Excavation: Robots are employed in mining and excavation activities where conditions might be unsafe for humans due to factors such as unstable terrain, toxic gases, or extreme temperatures.
5. Industrial Automation: AI-powered robots are used in industrial settings to handle dangerous manufacturing processes that involve heavy machinery, high temperatures, or exposure to toxic substances.
6. Underwater Exploration: Autonomous underwater robots equipped with AI systems can explore deep-sea environments, conduct research, and inspect underwater structures without putting human divers at risk.
7. Space Exploration: Robots equipped with AI are utilized in space missions to perform tasks on distant planets or in space where human presence is not feasible or safe.
8. Surveillance and Security: AI-powered robots can be used for surveillance and security in dangerous areas, such as border patrolling, monitoring critical infrastructure, and tracking potential threats.
9. Agricultural Tasks: In some agricultural settings, robots equipped with AI can handle dangerous tasks like pesticide spraying, which may involve exposure to harmful chemicals.
10. Medical Applications: AI-powered surgical robots can assist surgeons in complex procedures, improving precision and minimizing risks to patients.
These robots utilize various AI technologies such as machine learning, computer vision, natural language processing, and autonomous navigation to perceive their surroundings, make decisions based on the data they collect, and perform tasks effectively and safely. The use of AI in robots enables them to adapt to changing conditions, learn from experiences, and operate with a level of autonomy that makes them valuable tools in mitigating risks and ensuring the safety of human workers.
#14 AI-driven virtual reality and augmented reality applications offer immersive experiences in gaming industries.
AI-driven virtual reality (VR) and augmented reality (AR) applications offer immersive experiences in gaming industries through the integration of artificial intelligence and advanced algorithms with VR/AR technologies. Here’s how AI enhances and enriches these experiences:
1. Realistic environments: AI can generate highly realistic and detailed virtual environments by using techniques like procedural generation and deep learning. This allows game developers to create vast and lifelike virtual worlds that players can explore and interact with, enhancing the overall immersion.
2. Natural interactions: AI enables more natural and intuitive interactions between players and the virtual environment. Through computer vision and gesture recognition, players can use hand movements or voice commands to interact with objects and characters in the game world, making the experience more engaging.
3. Intelligent NPCs: AI-driven non-player characters (NPCs) can exhibit more realistic and dynamic behavior, making them feel more like real beings rather than scripted entities. These NPCs can adapt to the player’s actions, respond to their emotions, and even learn from their behavior, creating a more compelling gaming experience.
4. Personalized gameplay: AI algorithms can analyze player behavior and preferences to tailor the gaming experience to each individual. This personalization can include adjusting the difficulty level, presenting relevant challenges, and offering content that aligns with the player’s interests, maximizing enjoyment.
5. Adaptive storytelling: AI can dynamically alter the game’s narrative based on the player’s choices and actions, creating a unique and personalized storyline. This feature enhances the replayability of games, as each playthrough can lead to different outcomes and experiences.
6. Real-time analytics: AI can collect and analyze vast amounts of player data in real-time, providing insights to game developers and publishers. This data-driven approach helps identify player patterns, preferences, and pain points, allowing for continuous improvements and updates to enhance the gaming experience.
7. Natural language processing: In VR/AR games, players can engage in conversations with virtual characters through voice commands. AI-powered natural language processing enables these characters to understand and respond to spoken language, making interactions feel more natural and immersive.
8. AI-generated content: AI can assist game developers in generating various elements of the game, such as textures, characters, and environments. This reduces the need for manual content creation and expedites the game development process while maintaining a high level of quality.
Overall, the combination of AI and VR/AR technologies opens up new possibilities for creating immersive, interactive, and captivating gaming experiences that push the boundaries of traditional gaming and storytelling. As AI continues to advance, we can expect even more groundbreaking applications and experiences in the gaming industry.
#15 AI enhances weather forecasting
AI has significantly enhanced weather forecasting by leveraging advanced algorithms and computational power to analyze vast amounts of data and make more accurate predictions. Here are some ways AI enhances weather forecasting:
1. Data assimilation: Weather forecasting relies on various sources of data, such as satellite imagery, radar data, weather stations, and atmospheric measurements. AI algorithms can assimilate and process this data rapidly, combining it with historical data to improve the accuracy of weather models.
2. Improved models: AI techniques, including machine learning and neural networks, are used to develop more sophisticated and complex weather models. These models can better simulate the Earth’s atmosphere and weather patterns, leading to more precise forecasts.
3. Pattern recognition: AI can detect subtle patterns and correlations in weather data that human forecasters might miss. By recognizing these patterns, AI algorithms can provide insights into the development of weather systems and their potential impacts.
4. Ensemble forecasting: AI allows weather models to generate multiple forecasts, known as ensemble forecasts. By considering multiple possible outcomes, forecasters can better understand the uncertainty and probability of different weather scenarios, improving risk assessment and preparedness.
5. Nowcasting: AI enables “nowcasting,” which is short-term weather forecasting that provides real-time updates on rapidly changing weather conditions. By processing live data from various sources, AI can produce highly localized and accurate forecasts for the next few hours.
6. Extreme event prediction: AI models can identify conditions that may lead to extreme weather events, such as hurricanes, tornadoes, or heatwaves. This capability enhances early warning systems and helps authorities and the public take necessary precautions to minimize the impact of such events.
7. Data analysis speed: AI’s ability to analyze vast amounts of data quickly allows meteorologists to process and interpret information faster than traditional methods. This speed is crucial for time-sensitive weather events and emergency responses.
8. Personalized forecasting: AI can analyze individual user data and provide personalized weather forecasts based on location, preferences, and historical weather patterns. This level of personalization enhances the user experience and helps people plan their activities more effectively.
9. Continuous learning and improvement: AI systems can continuously learn from new data and update their forecasting models accordingly. This adaptability ensures that weather forecasts become more accurate over time.
Overall, AI’s ability to process, analyze, and interpret large volumes of data in real-time has revolutionized weather forecasting, making it more reliable, accessible, and valuable in various sectors, including agriculture, transportation, disaster management, and public safety.
#16 AI-powered smart homes control devices
AI-powered smart homes control devices through a combination of hardware, software, and artificial intelligence algorithms. Here’s a general overview of how it works:
1. **Internet of Things (IoT) Devices**: Smart homes are equipped with a variety of IoT devices such as smart bulbs, thermostats, security cameras, smart plugs, smart speakers, and more. These devices are designed to connect to the internet and communicate with each other, as well as with a central hub or a cloud-based platform.
2. **Central Hub or Cloud Platform**: In most cases, smart homes are controlled through a central hub or a cloud-based platform. The central hub can be a smart speaker (e.g., Amazon Echo, Google Home) or a dedicated smart home controller (e.g., SmartThings hub). Alternatively, some systems operate through cloud platforms that facilitate communication between devices and user interactions.
3. **Data Collection and Analysis**: The IoT devices in a smart home continuously collect data from their surroundings and send it to the central hub or cloud platform. For example, a smart thermostat can gather temperature and humidity data, while a smart camera can capture video and audio.
4. **Artificial Intelligence Algorithms**: The AI algorithms come into play when processing the data collected from the IoT devices. These algorithms use machine learning and data analysis techniques to understand patterns, user preferences, and behavior. Over time, the AI system becomes more intelligent and personalized as it learns from user interactions and historical data.
5. **Voice Commands and User Interface**: One of the key features of AI-powered smart homes is their ability to understand and respond to voice commands. Users can interact with their smart home devices by speaking to the central hub or through mobile apps that connect to the cloud platform. AI-powered voice assistants like Amazon’s Alexa and Google Assistant interpret these voice commands and carry out the appropriate actions.
6. **Automation and Control**: Once the AI system understands user preferences and patterns, it can automate tasks based on predefined rules or user-specific commands. For example, the system can adjust the thermostat based on the user’s preferred temperature settings, turn on the lights when someone enters a room, or set the coffee maker to start brewing at a certain time in the morning.
7. **Remote Access**: AI-powered smart homes also offer remote access, allowing users to control their devices and monitor their homes from anywhere with an internet connection. This can be done through dedicated mobile apps or web interfaces provided by the manufacturer or third-party platforms.
8. **Security and Privacy**: AI-powered smart home systems typically implement security measures to protect user data and privacy. Encryption, user authentication, and secure communication protocols are commonly used to ensure that unauthorized individuals cannot access or control smart devices.
In summary, AI-powered smart homes control devices by using AI algorithms to analyze data collected from IoT devices, interpreting user commands (especially through voice interactions), and automating tasks to create a more convenient and personalized living environment.
#17 AI algorithms improve personalized marketing strategies
AI algorithms have significantly improved personalized marketing strategies in various ways. Here are some key ways in which AI algorithms contribute to enhancing personalized marketing:
1. **Data analysis and segmentation:** AI algorithms can efficiently process vast amounts of data, including customer demographics, behavior, preferences, purchase history, and online interactions. By analyzing this data, AI can segment customers into distinct groups based on shared characteristics and behaviors. This segmentation allows marketers to tailor their messages and offers to specific customer segments, increasing the relevance of marketing efforts.
2. **Recommendation engines:** AI-powered recommendation engines use machine learning algorithms to analyze customer data and predict their preferences. These algorithms can suggest products, services, or content that are most likely to resonate with individual customers. Personalized product recommendations lead to higher conversion rates and increased customer satisfaction.
3. **Predictive analytics:** AI can predict future customer behavior and buying patterns based on historical data. This allows marketers to anticipate customer needs, identify potential churn, and proactively engage with customers before they make a decision. By targeting customers with personalized offers at the right time, businesses can increase customer retention and boost sales.
4. **Content personalization:** AI algorithms can analyze customer interactions with content, such as website visits, clicks, and engagement metrics, to understand individual preferences. Marketers can then deliver personalized content to customers based on their interests, ensuring a more engaging experience and increasing the likelihood of conversion.
5. **Chatbots and virtual assistants:** AI-powered chatbots and virtual assistants can interact with customers in real-time, providing personalized responses and recommendations. These AI-driven conversational interfaces can help customers find products, answer questions, and resolve issues efficiently, creating a more personalized and positive customer experience.
6. **A/B testing optimization:** AI algorithms can optimize A/B testing processes by quickly analyzing and identifying winning variations. This allows marketers to test multiple personalized marketing approaches simultaneously, refining their strategies based on real-time feedback and maximizing results.
7. **Dynamic pricing:** AI algorithms can analyze market conditions, customer behavior, and competitor pricing to adjust product prices in real-time. By offering personalized discounts or pricing based on individual customer preferences, businesses can increase conversions and loyalty.
8. **Social media targeting:** AI algorithms can analyze social media behavior and sentiment to identify potential customers who match specific criteria or exhibit interest in particular products or topics. This enables marketers to target their social media advertising to the most relevant audiences, improving campaign effectiveness.
9. **Customer service personalization:** AI algorithms can analyze customer service interactions and historical data to provide personalized support and assistance. By understanding individual preferences and past interactions, AI-powered customer service systems can deliver more tailored and effective solutions.
In summary, AI algorithms improve personalized marketing strategies by leveraging data analysis, predictive capabilities, and automation to deliver highly relevant and targeted experiences to customers. This not only enhances customer satisfaction and loyalty but also helps businesses achieve better results and higher returns on their marketing investments.
#18 AI optimizes supply chain management
AI optimizes supply chain management through various techniques and applications that leverage data, machine learning, and advanced analytics. Here are some ways AI can enhance supply chain management:
1. Demand forecasting: AI algorithms can analyze historical sales data, market trends, weather patterns, and other relevant factors to generate more accurate demand forecasts. This enables companies to better plan their inventory levels, avoid stockouts, and reduce excess inventory costs.
2. Inventory management: AI can help optimize inventory levels by predicting demand fluctuations and suggesting the right reorder points. It can also identify slow-moving or obsolete items, allowing businesses to make informed decisions about inventory allocation and reduce carrying costs.
3. Route optimization: AI-powered algorithms can optimize transportation routes and delivery schedules, considering factors like traffic conditions, weather, and real-time updates. This helps minimize delivery time, fuel consumption, and transportation costs.
4. Supplier selection and risk assessment: AI can assess supplier performance, track supplier data, and identify potential risks in the supply chain. By analyzing various data points, including financial health, quality metrics, and delivery history, AI can assist in selecting reliable suppliers and mitigating potential disruptions.
5. Warehouse automation: AI-powered robotics and automation technologies can streamline warehouse operations. These technologies can manage inventory, handle picking and packing tasks, and optimize storage layouts for better space utilization.
6. Quality control and predictive maintenance: AI can monitor production processes and detect anomalies in real-time, enabling proactive quality control. It can also predict equipment failures and maintenance needs, reducing downtime and enhancing overall operational efficiency.
7. Cost optimization: AI algorithms can analyze supply chain data to identify cost-saving opportunities, such as sourcing alternatives, transportation optimizations, and energy efficiency improvements.
8. Risk management: AI can help identify potential risks and vulnerabilities in the supply chain, such as geopolitical issues, natural disasters, or supplier disruptions. This allows businesses to implement contingency plans and build more resilient supply chains.
9. Personalized customer experiences: AI can analyze customer data to provide personalized product recommendations and delivery options, enhancing customer satisfaction and loyalty.
10. Real-time visibility and analytics: AI-powered analytics platforms provide real-time visibility into the entire supply chain, allowing businesses to identify bottlenecks, anticipate issues, and make data-driven decisions promptly.
By leveraging AI technologies in these ways, companies can optimize their supply chain operations, reduce costs, improve efficiency, and respond more effectively to changing market dynamics.
#19 AI aids in drug discovery and medical research
AI plays a crucial role in drug discovery and medical research by leveraging its capabilities in data analysis, pattern recognition, and predictive modeling. Here are some ways in which AI aids in these fields:
1. **Data Analysis and Integration:** Drug discovery and medical research generate vast amounts of data from various sources, including clinical trials, genetic studies, electronic health records, and scientific literature. AI can process and analyze this data, integrating information from different sources to identify patterns and potential correlations that humans might miss.
2. **Target Identification and Validation:** Identifying potential drug targets is a critical step in drug discovery. AI can analyze biological and genetic data to pinpoint molecules, proteins, or genes that might play a role in a particular disease. By validating these targets computationally, researchers can prioritize which ones to pursue further.
3. **Virtual Screening of Compounds:** AI algorithms can efficiently perform virtual screening of large databases containing millions of chemical compounds. This helps identify potential drug candidates that could interact with the chosen target, saving time and resources compared to traditional experimental methods.
4. **Drug Design and Optimization:** AI can assist in designing new drugs or optimizing existing ones by predicting their interactions with biological targets. This allows researchers to create molecules with desired properties and increase the likelihood of success in clinical trials.
5. **Personalized Medicine:** AI can analyze patient data, such as genetic information and medical history, to tailor treatments to an individual’s specific needs. This enables personalized medicine, where therapies are customized for each patient, leading to better outcomes and reduced side effects.
6. **Clinical Trial Optimization:** AI can help optimize clinical trial design by identifying suitable patient cohorts, predicting patient responses, and even simulating trial outcomes under various conditions. This streamlines the drug development process and improves the chances of successful trials.
7. **Drug Repurposing:** AI can identify potential new uses for existing drugs by analyzing their interactions with different biological targets. This approach, known as drug repurposing, can speed up the development of treatments for new indications.
8. **Natural Language Processing (NLP) in Medical Literature:** AI-powered NLP tools can quickly sift through vast amounts of scientific literature, extracting relevant information, and summarizing findings. This helps researchers stay up-to-date with the latest developments and form hypotheses for further investigation.
9. **Image Analysis and Diagnostics:** AI excels at image analysis, making it valuable in medical imaging applications such as radiology and pathology. AI algorithms can assist in diagnosing diseases, identifying abnormalities, and predicting patient outcomes based on medical images.
Overall, AI’s ability to analyze complex data, discover patterns, and make predictions has significantly accelerated drug discovery and medical research, leading to more effective treatments and improved patient care.
#20 AI assists in personalized fashion recommendations
AI can assist in personalized fashion recommendations through the application of machine learning algorithms and data analysis. The process typically involves the following steps:
1. Data Collection: AI systems gather relevant data about the user’s preferences, body measurements, fashion style, browsing history, and any other relevant information. This data can be collected through surveys, questionnaires, user interactions on fashion websites, and other sources.
2. Profile Creation: Based on the collected data, AI creates a unique profile for each user. This profile includes information about the user’s body type, preferred colors, clothing sizes, style preferences, favorite brands, and other relevant details.
3. Collaborative Filtering: AI uses collaborative filtering techniques to identify patterns and similarities between different users with similar preferences. It looks for users who have exhibited similar fashion tastes and recommends items based on what others with similar profiles have liked or purchased.
4. Content Analysis: AI systems analyze fashion-related content, including images, product descriptions, and customer reviews, to understand the characteristics of various clothing items and accessories. This allows AI to match products more accurately to users’ preferences.
5. Natural Language Processing (NLP): NLP enables AI to understand and process textual data, such as user feedback, reviews, and product descriptions. This helps in better understanding user preferences and making more relevant recommendations.
6. Image Recognition: AI can incorporate image recognition capabilities to analyze visual data, such as outfit photos and style inspirations. By identifying patterns and trends in these images, AI can offer personalized suggestions based on the user’s visual preferences.
7. Continuous Learning: AI systems are designed to learn and improve over time by incorporating user feedback and interactions. As users provide feedback on the recommended items, the AI adapts its recommendations to better suit individual preferences.
8. Contextual Information: AI can take into account contextual factors like weather, occasion, and location to offer more relevant and practical fashion suggestions.
9. A/B Testing: AI can perform A/B testing, where different recommendations are presented to different users, and their responses are measured to determine which recommendations are more effective and well-received.
10. Integration with Retailers: AI systems can integrate with fashion retailers, e-commerce platforms, and fashion apps, allowing seamless access to a wide range of clothing items and accessories. This enables users to purchase the recommended products directly through the AI-powered platform.
By combining these techniques, AI provides personalized fashion recommendations that cater to the unique preferences and styles of individual users, making the shopping experience more convenient, enjoyable, and satisfying.
#21 AI-driven language processing helps with grammar and spell-checking
AI-driven language processing helps with grammar and spell-checking through various mechanisms and techniques. Here’s how it works:
1. **Natural Language Processing (NLP)**: AI-driven language processing relies on NLP algorithms to understand and analyze human language. NLP enables the system to comprehend the context, syntax, and semantics of a sentence or text.
2. **Language Models**: AI language models, like GPT-3.5, are trained on vast amounts of text data, which enables them to learn the patterns and rules of language. These models can predict the most likely words, phrases, and sentence structures based on the input they receive.
3. **Grammar Rules and Patterns**: Language processing systems use a set of predefined grammar rules and patterns to check for grammatical errors. For example, they can identify subject-verb agreement errors, incorrect verb tense, misplaced modifiers, and more.
4. **Spell Checking**: AI language processors incorporate dictionaries and language databases to detect and correct spelling mistakes. They compare words in the text against a vast database of correctly spelled words and suggest corrections for any mismatches.
5. **Contextual Understanding**: One of the most significant advantages of AI-driven language processing is its ability to understand the context of a sentence. It considers the surrounding words and phrases to determine the correct interpretation and make appropriate suggestions.
6. **Machine Learning**: AI-powered language processing systems often use machine learning algorithms to improve their performance over time. They can learn from user interactions, user corrections, and updates to the language to become more accurate and adaptive in identifying errors.
7. **Error Suggestions**: When an error is detected, the language processing system can offer suggestions to correct the mistake. These suggestions are generated based on the most probable corrections derived from the model’s training data.
8. **Personalization**: Some AI-driven language processors can be personalized to adapt to an individual’s writing style and specific language preferences. This feature enhances the accuracy of suggestions and corrections.
9. **Real-Time Feedback**: Many language processing tools provide real-time feedback as users type, underlining or highlighting potential errors. This allows users to correct mistakes on the spot and improve their writing as they go.
Overall, AI-driven language processing combines NLP, machine learning, grammar rules, and vast language datasets to provide accurate and efficient grammar and spell-checking capabilities, making it a valuable tool for writers, students, professionals, and anyone looking to enhance their writing skills.
#22 AI-powered recommendation systems facilitate better job matching in recruitment processes
AI-powered recommendation systems can significantly enhance the job matching process in recruitment by leveraging various techniques and data sources to make more accurate and efficient recommendations. Here’s how they work:
1. **Candidate Profiling**: AI-powered recommendation systems start by creating detailed profiles of both candidates and job openings. For candidates, the system analyzes their resumes, cover letters, and other relevant data to understand their skills, qualifications, work experience, education, and preferences. For job openings, the system assesses the job descriptions, required skills, and other essential attributes.
2. **Data Analysis**: These recommendation systems use advanced algorithms to analyze vast amounts of historical data from previous successful job matches. They can learn patterns and correlations between candidate profiles and job requirements, enabling them to identify crucial factors that contribute to a successful match.
3. **Machine Learning Algorithms**: AI-powered recommendation systems utilize machine learning techniques to continuously improve their accuracy. As they process more data and receive feedback on previous recommendations, they can fine-tune their algorithms to make more relevant and personalized suggestions.
4. **Semantic Understanding**: Advanced recommendation systems go beyond keyword matching. They use natural language processing (NLP) and semantic understanding to comprehend the context and meaning of job descriptions and candidate profiles. This enables a deeper understanding of candidate skills and job requirements, leading to more precise matching.
5. **Skill Gaps Analysis**: AI can assess the skills required for a particular job and compare them to the skills possessed by candidates. By identifying skill gaps, the system can recommend candidates who possess most, if not all, of the essential skills needed for the job.
6. **Behavioral Analysis**: Some recommendation systems can analyze candidates’ online behavior, such as their interactions with job postings and company websites. This analysis can offer insights into their level of interest and motivation for a specific job, further improving the matching process.
7. **Continuous Learning**: AI-powered recommendation systems continuously learn and adapt based on new data and changing job market trends. This adaptability ensures that the recommendations stay up-to-date and relevant over time.
8. **Reduction of Bias**: By relying on data-driven insights, AI-powered systems have the potential to reduce unconscious biases that can affect the hiring process. They focus on objective criteria and qualifications, thereby promoting fairer and more inclusive job matches.
9. **Time and Cost Efficiency**: Recruitment processes can be time-consuming and resource-intensive. AI-powered recommendation systems can significantly speed up the screening and matching process, leading to faster candidate shortlisting and ultimately reducing time-to-hire.
10. **Personalization**: These systems can provide personalized recommendations to both candidates and employers, increasing the likelihood of finding the right fit for both parties.
By combining data analysis, machine learning, semantic understanding, and continuous learning, AI-powered recommendation systems can revolutionize the recruitment process, leading to better job matching and improved outcomes for both job seekers and employers.
#23 AI enhances cybersecurity
AI enhances cybersecurity in several ways by leveraging its capabilities to detect, prevent, and respond to cyber threats more effectively than traditional methods. Some of the key ways AI enhances cybersecurity are:
1. **Threat Detection and Analysis:** AI-powered cybersecurity systems can analyze vast amounts of data from various sources, such as network logs, user behavior, and system activities, to detect patterns indicative of cyber threats. Machine learning algorithms can identify anomalies and potential security breaches, enabling faster and more accurate threat detection.
2. **Real-Time Monitoring and Incident Response:** AI can continuously monitor network activities in real-time. It can detect and respond to suspicious activities promptly, minimizing the time between a security incident occurring and its mitigation. AI-based systems can also automate incident response procedures, improving overall response times.
3. **Behavioral Analysis:** AI can analyze user behavior to create baselines for normal activities. Any deviations from these baselines can trigger alerts, indicating potential insider threats or unauthorized access attempts.
4. **Advanced Malware Detection:** AI algorithms can analyze the characteristics of known malware and identify new malware variants based on similarities. This proactive approach helps in detecting and blocking zero-day threats that haven’t been seen before.
5. **Phishing and Fraud Detection:** AI can analyze emails, websites, and other communication channels to identify phishing attempts and fraudulent activities. It can recognize patterns commonly associated with phishing and warn users or block malicious content.
6. **Vulnerability Assessment:** AI can assist in identifying vulnerabilities in software and systems by analyzing code, configurations, and network setups. This helps organizations prioritize and patch critical vulnerabilities quickly.
7. **User Authentication and Access Control:** AI-based authentication systems can analyze user behavior, biometric data, and contextual information to verify users’ identities more securely and accurately than traditional methods like passwords.
8. **Security Automation:** AI enables security automation, reducing the manual effort required to respond to alerts and security incidents. Automated processes can quarantine infected systems, block malicious traffic, and update security policies in real-time.
9. **Predictive Analytics:** AI can predict potential security threats based on historical data and ongoing trends. This capability allows organizations to take proactive measures to prevent cyber attacks.
10. **Adaptive Security:** AI can adapt and learn from new threats and attack patterns. As cybercriminals develop more sophisticated techniques, AI can evolve its defense strategies to stay ahead of potential threats.
11. **Reducing False Positives:** AI can refine its analysis over time, reducing the number of false positives that traditional security systems may produce. This helps security teams focus on genuine threats and improve operational efficiency.
However, it’s important to note that while AI significantly enhances cybersecurity, it is not a standalone solution. It works best when combined with human expertise and a holistic cybersecurity strategy that includes regular updates, patches, employee training, and other cybersecurity best practices.
#24 AI-driven agriculture technology optimizes crop yield
AI-driven agriculture technology optimizes crop yield through the application of advanced analytics, machine learning, and data-driven insights. Here’s how it works:
1. **Data Collection:** The process begins with data collection from various sources. This includes information on weather patterns, soil quality, historical crop yields, satellite imagery, and other relevant data points. Drones and IoT devices are often used to gather real-time data from the fields.
2. **Data Analysis:** AI algorithms process and analyze the collected data to identify patterns, trends, and correlations. For example, they can determine how different weather conditions impact crop growth, how specific soil compositions affect plant health, and how pests or diseases spread.
3. **Precision Farming:** AI enables precision farming techniques, where the farmer can apply resources such as water, fertilizers, and pesticides with pinpoint accuracy based on the data analysis. This reduces waste and ensures that crops receive exactly what they need for optimal growth.
4. **Crop Monitoring:** AI-powered sensors and cameras continuously monitor crops, assessing their health and growth stages. If any issues are detected, such as pest infestations or nutrient deficiencies, the technology can alert farmers promptly, enabling them to take immediate action.
5. **Predictive Analytics:** AI can make predictions about crop growth, yield potential, and market demand. This information helps farmers plan better and make informed decisions about crop selection, planting schedules, and harvesting times.
6. **Automated Machinery:** AI can be integrated into agricultural machinery and robots to perform tasks like planting, harvesting, and weeding with greater precision and efficiency.
7. **Disease Detection:** AI algorithms can quickly identify signs of disease or stress in crops, allowing farmers to address the issues before they spread and cause significant damage.
8. **Crop Breeding:** AI can accelerate crop breeding programs by analyzing genetic data to identify desirable traits and crossbreeding strategies that lead to higher yields and resilience against environmental challenges.
9. **Recommendation Systems:** AI-driven agriculture platforms can provide personalized recommendations to farmers based on their specific fields and crops, offering insights on optimal planting methods, pest control strategies, and crop rotation plans.
10. **Optimization Algorithms:** AI can create optimized planting and irrigation schedules based on historical data and future predictions, leading to better resource management and increased crop yield.
By combining data-driven insights, real-time monitoring, predictive capabilities, and precision farming techniques, AI-driven agriculture technology helps farmers make informed decisions and implement best practices that ultimately lead to higher crop yields and improved overall agricultural productivity.
#25 AI-powered drones assist in disaster management
AI-powered drones play a crucial role in disaster management by providing valuable support and capabilities that aid in various stages of disaster response and recovery. Here are some ways in which AI-powered drones assist in disaster management:
1. Aerial assessment and mapping: Drones equipped with AI-enabled cameras and sensors can quickly fly over disaster-stricken areas, capturing high-resolution images and data. AI algorithms can then analyze this data to create detailed maps, identify damaged infrastructure, assess the extent of the disaster, and locate areas that need immediate attention.
2. Search and rescue operations: Drones equipped with thermal imaging and AI-driven object recognition can assist in locating survivors and missing persons in disaster zones. They can cover large areas much faster than ground-based teams, improving the chances of finding people in need of help.
3. Communication and connectivity: In some disasters, communication infrastructure may be severely affected. AI-powered drones can be used as flying communication relays to restore connectivity by establishing temporary networks and providing a means for affected communities to communicate with rescue teams and authorities.
4. Hazard assessment: AI-powered drones can be equipped with gas and chemical sensors to identify hazardous materials or leaks, which can help responders understand potential risks and devise appropriate strategies for handling the situation safely.
5. Damage assessment and infrastructure inspection: After a disaster, AI-powered drones can conduct thorough inspections of critical infrastructure, such as bridges, buildings, and power lines, to assess damage and identify structural weaknesses. This information is invaluable for planning recovery efforts and ensuring the safety of response teams.
6. Environmental monitoring: Drones equipped with AI can monitor environmental factors such as air and water quality, temperature, and radiation levels in disaster-affected areas. This information aids in understanding the impact of the disaster on the environment and helps authorities make informed decisions regarding public health and environmental concerns.
7. Predictive analytics: AI algorithms can process data collected from drones and other sources to generate predictive models for future disasters. This information helps disaster management agencies prepare and plan more effectively for potential hazards.
8. Autonomous operations: AI-powered drones can be programmed to operate autonomously, making them efficient and capable of handling repetitive tasks without direct human control. This frees up human responders to focus on more complex and critical aspects of disaster management.
By leveraging AI technologies, drones become versatile tools that significantly enhance disaster management capabilities. They enable rapid response, improve situational awareness, and increase the efficiency and effectiveness of rescue and recovery efforts, ultimately saving lives and reducing the impact of disasters on affected communities.
#26 AI enhances the gaming industry
AI has indeed significantly enhanced the gaming industry in various ways. Its integration has led to improved gameplay experiences, enhanced graphics, smarter NPCs (non-playable characters), better game design, and more efficient game development processes. Here are some specific ways AI enhances the gaming industry:
1. **Realistic graphics and physics:** AI-powered algorithms, such as deep learning techniques, have been employed to enhance graphics and physics simulations in games. This has resulted in more realistic visuals, lifelike animations, and immersive environments.
2. **Procedural content generation:** AI can generate content procedurally, meaning it can create game elements like levels, maps, characters, and even narratives automatically. This approach helps in reducing development time and keeps the game fresh for players with dynamic and ever-changing content.
3. **Improved gameplay and adaptive difficulty:** AI algorithms can analyze player behavior and adapt the difficulty level accordingly. This ensures that players are continually challenged but not overwhelmed, leading to a more enjoyable and engaging experience.
4. **Smart NPCs:** AI allows game developers to create more intelligent and dynamic non-playable characters (NPCs). NPCs can learn from player interactions, make decisions based on the game’s context, and offer more realistic and challenging opponents.
5. **Natural language processing (NLP) and voice recognition:** AI-powered NLP and voice recognition technologies enable more interactive and natural player interactions. Players can communicate with NPCs or control in-game actions using voice commands, making the gaming experience more immersive.
6. **Game testing and bug detection:** AI can help in identifying bugs and glitches in games more efficiently than manual testing. Machine learning models can be trained to detect anomalies and provide developers with insights into potential issues.
7. **Personalized gaming experiences:** AI can analyze player preferences, behaviors, and habits to provide personalized gaming experiences. This includes recommending games based on individual tastes or adapting in-game content to suit a player’s style.
8. **AI-generated content and storytelling:** AI algorithms are being used to generate narratives and storylines for games. This can lead to dynamic and evolving stories that change based on player choices and actions.
9. **Player behavior analysis:** Game companies can use AI to analyze player data and gain valuable insights into player behavior and preferences. This information helps in refining games, designing targeted marketing strategies, and making data-driven decisions.
10. **Game design assistance:** AI can assist game developers in various aspects of the design process, from concept art generation to balancing gameplay mechanics. This streamlines the development process and fosters innovation.
In summary, AI has revolutionized the gaming industry by providing developers with powerful tools to create more immersive, engaging, and innovative gaming experiences. It continues to play a crucial role in shaping the future of the gaming world, offering endless possibilities for both developers and players alike.
#27 AI algorithms analyze social media data
AI algorithms have become integral to the analysis of social media data, revolutionizing the way businesses, researchers, and individuals comprehend and utilize the vast amount of information generated on various social media platforms. With the explosive growth of social media usage, the volume and diversity of data have surpassed human capacity to manually process it effectively. AI algorithms step in to address this challenge, bringing significant advantages to social media analysis.
1. **Data Collection and Processing**: AI algorithms can efficiently gather and process enormous amounts of data from social media platforms. They can crawl through various sources, extract relevant information, and organize it into structured datasets. Natural Language Processing (NLP) techniques are used to understand and interpret textual data, while Computer Vision algorithms handle image and video content.
2. **Sentiment Analysis**: One of the essential applications of AI in social media analysis is sentiment analysis. By employing NLP and machine learning, AI algorithms can determine the sentiment (positive, negative, neutral) behind social media posts, comments, and interactions. This information is valuable for understanding public opinion, measuring customer satisfaction, and gauging brand reputation.
3. **Trend and Topic Detection**: AI algorithms can identify emerging trends and popular topics on social media. By analyzing hashtags, keywords, and user engagement patterns, they can pinpoint the most discussed subjects, helping businesses and marketers stay ahead of the curve in their campaigns and strategies.
4. **User Behavior Analysis**: AI algorithms analyze user behavior on social media platforms, providing valuable insights into how people interact with content. This information helps businesses tailor their content to suit their target audience and improve engagement levels.
5. **Social Listening and Customer Insights**: AI-powered social listening tools enable organizations to monitor mentions and discussions about their brand, products, or services in real-time. By analyzing this data, companies can gain valuable customer insights, understand pain points, and identify areas for improvement.
6. **Influencer Marketing**: AI algorithms assist in identifying influencers whose audience aligns with a brand’s target demographics. By analyzing the followers, engagement rates, and content of potential influencers, AI helps businesses make informed decisions about their influencer marketing strategies.
7. **Fake News and Misinformation Detection**: With the proliferation of fake news and misinformation on social media, AI algorithms play a crucial role in identifying and flagging such content. By using pattern recognition and NLP, these algorithms help minimize the spread of false information.
8. **Personalized Content Recommendations**: Social media platforms leverage AI algorithms to deliver personalized content recommendations to users. By analyzing user behavior, preferences, and interactions, AI can present users with content that aligns with their interests, increasing engagement and user satisfaction.
9. **Social Media Advertising**: AI-driven ad targeting ensures that advertisements reach the most relevant audiences. Algorithms analyze user data to create detailed profiles, allowing businesses to deliver targeted ads based on demographics, interests, and online behaviors.
10. **Crisis Monitoring and Public Opinion**: During crises and significant events, AI algorithms can monitor social media conversations to gauge public sentiment and reactions. This information helps governments, organizations, and emergency responders make informed decisions and respond effectively.
However, it’s essential to acknowledge the ethical implications of AI in social media data analysis. Privacy concerns, data security, and potential biases in AI algorithms must be carefully addressed to ensure responsible and transparent use of AI technology in social media analysis. As AI continues to advance, it is expected to play an even more prominent role in shaping the future of social media and its impact on society.
#28 AI-driven language learning apps improve language acquisition through personalized exercises
AI-driven language learning apps can indeed enhance language acquisition through personalized exercises by leveraging the capabilities of artificial intelligence. Here’s how they achieve this:
1. **Adaptive Learning:** These apps use AI algorithms to analyze the learner’s strengths and weaknesses. They assess the learner’s language proficiency level, identify areas that need improvement, and then adapt the learning content accordingly. By tailoring the exercises to individual needs, learners can focus on areas where they need the most practice, maximizing their learning efficiency.
2. **Personalized Content:** AI-powered language learning apps can generate and curate content based on the learner’s interests, goals, and proficiency level. This means learners get exercises, texts, and activities that are relevant to their preferences, making the learning process more engaging and motivating.
3. **Intelligent Feedback:** AI can provide instant and detailed feedback on language exercises. Whether it’s grammar, pronunciation, or vocabulary, the AI algorithms can identify errors and provide explanations or suggestions for improvement. This real-time feedback helps learners correct mistakes immediately, reinforcing their understanding of the language rules.
4. **Natural Language Processing (NLP):** AI-driven apps often employ NLP to understand the learner’s spoken or written responses. NLP allows the app to interpret context and meaning, enabling more sophisticated interactions and a more human-like learning experience.
5. **Speech Recognition:** For language learning apps focused on speaking skills, AI-powered speech recognition technology can assess the learner’s pronunciation and fluency. This helps learners practice speaking in a more accurate and confident manner.
6. **Data-driven Insights:** AI gathers data on the learner’s progress and performance over time. This data can be analyzed to provide insights into the learner’s strengths, weaknesses, and overall progress. Teachers or the AI itself can use this information to customize future exercises and learning paths.
7. **Continuous Improvement:** AI algorithms continuously learn from user interactions and feedback. As more learners use the app, the AI model improves, making the exercises and feedback more accurate and effective over time.
8. **Gamification and Engagement:** Many AI-driven language learning apps incorporate gamification elements to keep learners engaged. These can include rewards, challenges, progress tracking, and social interactions. AI helps tailor these gamified elements to the individual learner’s preferences, making the experience more enjoyable and motivating.
Overall, AI-driven language learning apps offer personalized and dynamic learning experiences that cater to individual needs, preferences, and skill levels. By harnessing the power of AI, these apps aim to accelerate language acquisition and provide a more effective and enjoyable way to learn a new language.
#29 AI-powered home security systems offer real-time monitoring and alerts
AI-powered home security systems offer real-time monitoring and alerts through the integration of various technologies and intelligent algorithms. Here’s how they work:
1. **Security Cameras:** These systems use high-definition security cameras strategically placed around your home. These cameras can capture live video feeds and sometimes audio as well. Some cameras come with advanced features such as night vision, motion detection, and pan-tilt-zoom capabilities.
2. **Video Analytics:** AI algorithms are used to analyze the video feeds from the security cameras. Video analytics can detect and identify objects, people, and animals in the footage. The AI can also distinguish between normal events (e.g., a pet walking) and suspicious activities (e.g., an intruder).
3. **Motion Detection:** The AI system can detect motion in the camera’s field of view. This helps to trigger alerts when unexpected movements are detected, such as someone trying to enter your property or wandering around your home.
4. **Facial Recognition:** Some advanced home security systems employ facial recognition technology to identify known individuals, like family members or authorized guests. This can be used to customize access permissions or send specific alerts based on recognized faces.
5. **Object Recognition:** AI algorithms can recognize specific objects like vehicles, packages, or even weapons. This can be useful for tracking deliveries or identifying potential threats.
6. **Sound Analysis:** In addition to video, some systems also use sound analysis to detect specific events, such as breaking glass or the sound of smoke alarms going off, which could indicate a break-in or fire.
7. **Cloud Connectivity:** Many AI-powered security systems are cloud-based, meaning the data from the cameras and sensors are sent to a centralized server for processing and analysis. This allows for real-time monitoring and remote access to the system.
8. **Alerts and Notifications:** When the AI system identifies a potential threat or unusual activity, it sends immediate alerts to the homeowner’s smartphone or computer. These alerts can include push notifications, emails, or text messages, depending on the user’s preferences.
9. **Machine Learning:** Over time, the AI system can learn from its observations and user feedback. It can improve its accuracy in identifying suspicious events, reduce false alarms, and adapt to changing patterns of activity around the house.
By combining these technologies and AI capabilities, home security systems can offer real-time monitoring and alerts, providing homeowners with a heightened sense of security and peace of mind.
#30 AI facilitates financial planning and investment strategies
AI plays a significant role in facilitating financial planning and investment strategies in several ways. Here are some key ways in which AI contributes to these areas:
1. Data Analysis and Pattern Recognition: AI algorithms can process vast amounts of financial data quickly and efficiently. By analyzing historical market data, economic indicators, company financials, and various other relevant information, AI can identify patterns and trends that humans might miss. This helps in making more informed investment decisions.
2. Risk Assessment: AI can assess the risk associated with different investment options. By analyzing the historical volatility and correlations among various assets, AI can help investors build portfolios with an optimal risk-return trade-off based on their risk tolerance and financial goals.
3. Robo-Advisors: AI-powered robo-advisors are automated platforms that provide personalized investment advice and portfolio management. They use algorithms to understand an individual’s financial situation, goals, and risk tolerance to suggest appropriate investment strategies and asset allocations.
4. Natural Language Processing (NLP): AI can process and interpret large volumes of news articles, social media updates, and other textual data related to financial markets. NLP enables AI systems to gauge market sentiment, assess the impact of news events on specific assets, and make timely investment decisions based on sentiment analysis.
5. Algorithmic Trading: AI algorithms can execute trades based on predefined rules and market conditions without human intervention. High-frequency trading (HFT) and quantitative trading strategies rely heavily on AI and machine learning algorithms to identify profitable opportunities in the market.
6. Fraud Detection: AI is employed by financial institutions to detect and prevent fraudulent activities. Machine learning models can analyze transaction patterns and identify unusual behaviors, helping to protect customers’ assets.
7. Personalized Financial Planning: AI-powered financial planning tools can create personalized financial plans for individuals based on their income, expenses, and financial goals. These tools can also take into account changing life circumstances and adjust the plans accordingly.
8. Predictive Analytics: AI can forecast market movements and asset prices based on historical data and market indicators. While no prediction is foolproof, AI’s ability to analyze complex data can provide valuable insights for investors and traders.
9. Customer Service and Support: AI-driven chatbots and virtual assistants can handle customer queries, provide information about financial products, and offer support 24/7, improving customer experience and reducing response times.
Overall, AI’s ability to process vast amounts of data, identify patterns, and make data-driven decisions enables it to enhance financial planning and investment strategies by providing more accurate insights and improving decision-making processes. However, it’s essential to note that AI should be used as a tool in conjunction with human expertise and not as a replacement for human judgment in the financial domain.
#31 AI assists in content moderation, filtering out inappropriate and harmful content online
AI assists in content moderation by using advanced algorithms and machine learning techniques to analyze and filter out inappropriate and harmful content online. Here’s an overview of how this process typically works:
1. **Data Collection and Labeling**: The AI system is trained on a large dataset of content, including text, images, videos, and other multimedia, that has been labeled as either appropriate or inappropriate. Human moderators review and categorize this content, providing the AI with examples of what is considered acceptable and what should be filtered.
2. **Feature Extraction**: The AI system extracts relevant features from the data, which can vary depending on the type of content being analyzed. For text, this might involve breaking down sentences into words and understanding the context and sentiment. For images and videos, it could involve identifying objects, scenes, and visual cues.
3. **Machine Learning Model**: A machine learning model, such as a neural network, is trained using the labeled data and the extracted features. The model learns from the patterns present in the data and adjusts its parameters to improve its ability to distinguish between appropriate and inappropriate content.
4. **Real-Time Analysis**: Once the AI model is trained, it can be deployed to analyze content in real-time as it is uploaded or shared online. This process is often done in combination with human moderation to ensure greater accuracy and reduce false positives.
5. **User Feedback Loop**: As the AI system is deployed and used, it continuously learns from user feedback. When users report or flag content, the AI takes these reports into account to improve its accuracy over time.
6. **Filtering Mechanisms**: The AI system can be integrated into platforms and websites through filtering mechanisms. It can automatically flag or block content that is likely to be inappropriate or harmful, preventing it from being seen by the wider audience.
7. **Customization and Contextual Understanding**: Some AI content moderation systems allow customization to suit the specific needs and values of individual platforms or communities. They can also be designed to understand the context of the content, as some content might be appropriate in one context but not in another.
8. **Ongoing Maintenance and Updates**: Content moderation AI systems require regular maintenance and updates to keep up with evolving trends and new forms of inappropriate content. The AI model needs to be retrained periodically to stay effective in its task.
It’s essential to recognize that AI content moderation is not perfect and may still have limitations. Striking the right balance between automated filtering and human moderation remains a challenge, as some content might require nuanced understanding and cultural context that AI systems may struggle to grasp fully. However, AI plays a crucial role in scaling content moderation efforts and helping maintain safer online environments.
#32 AI improves voice recognition in transcription services, making it easier to convert speech to text
AI improves voice recognition in transcription services through a combination of advanced technologies and techniques. Here are some ways in which AI enhances voice recognition for more accurate and efficient speech-to-text conversion:
1. **Deep Learning Models**: AI systems leverage deep learning models, particularly recurrent neural networks (RNNs) and convolutional neural networks (CNNs), to process audio data. These models can learn complex patterns and representations from vast amounts of labeled training data, allowing them to better understand and interpret different speech patterns.
2. **Large Datasets**: AI systems require large and diverse datasets of audio recordings with corresponding transcriptions to train effectively. These datasets help AI models generalize and recognize different accents, speech variations, and speaking styles.
3. **Speech Preprocessing**: Prior to feeding the audio data into the AI model, it undergoes preprocessing steps such as noise reduction, filtering, and feature extraction. These steps help to enhance the clarity of the speech signal and extract relevant features that aid in transcription.
4. **Language Models**: AI-based voice recognition systems use language models to improve contextual understanding. Language models like Transformer-based architectures (e.g., BERT, GPT-3) can better comprehend the relationships between words and sentences, leading to more accurate transcriptions.
5. **Transfer Learning**: Transfer learning allows AI models to leverage knowledge gained from pretraining on a large dataset for a related task and then fine-tuning on a smaller, task-specific dataset. This approach helps boost performance even with limited data for the specific transcription task.
6. **Adaptation to Speaker Characteristics**: AI models can adapt to specific speaker characteristics over time. By collecting data from individual speakers and incorporating it into the training process, the system becomes more personalized and accurate for transcribing that speaker’s speech.
7. **Contextual Information**: AI systems can use contextual information from the surrounding sentences or paragraphs to make more accurate predictions for ambiguous speech segments. This helps in resolving homophones or words with similar phonetic sounds.
8. **Feedback Loop**: Transcription services that utilize AI often have a feedback loop that allows users to correct any mistakes made by the system. This feedback is used to continually improve the AI model and enhance its accuracy over time.
9. **Active Learning**: Some AI transcription systems incorporate active learning techniques, where the system identifies uncertain or challenging transcription instances and requests human intervention to provide accurate annotations. This helps in building a more robust training dataset.
10. **Continuous Improvement**: As more users interact with the transcription service, the system continues to learn from new data, leading to continuous improvement in accuracy and performance.
By integrating these AI-driven techniques and technologies, voice recognition in transcription services becomes more sophisticated, enabling more reliable and efficient conversion of speech to text.
#33 . AI enables predictive maintenance in industries
AI enables predictive maintenance in industries through the use of advanced data analytics and machine learning algorithms. Here’s how it works:
1. Data Collection: The first step in predictive maintenance is collecting relevant data from the industrial equipment and machinery. Sensors and IoT devices are often used to monitor various parameters such as temperature, pressure, vibration, humidity, and more. This data is continuously gathered and transmitted to a centralized database.
2. Data Preprocessing: The collected data may contain noise, outliers, or missing values. Before using it for predictive maintenance, preprocessing techniques are applied to clean and normalize the data, ensuring its quality and consistency.
3. Data Storage and Management: The data is stored in a structured format that allows easy retrieval and analysis. Cloud-based solutions are commonly used for scalable storage and easy access from multiple locations.
4. Feature Engineering: Engineers and data scientists identify relevant features and patterns in the data that can be used to predict potential failures. These features can be calculated based on the raw sensor data or other domain-specific knowledge.
5. Machine Learning Model Development: Various machine learning algorithms are trained on historical data to learn the patterns associated with equipment failures. These algorithms can be regression models, decision trees, random forests, support vector machines, neural networks, or other techniques suitable for the specific problem.
6. Model Training and Optimization: The selected machine learning models are trained on the preprocessed data. During training, the models adjust their internal parameters to minimize prediction errors. Hyperparameter tuning and cross-validation are performed to optimize the model’s performance.
7. Real-time Monitoring: The trained model is deployed in real-time to monitor the streaming data from the industrial equipment. It continuously analyzes the incoming data to identify any anomalies or signs of potential failure.
8. Anomaly Detection: When the AI model detects anomalies or deviations from normal operating conditions, it raises alerts to notify maintenance personnel. These alerts can be prioritized based on the severity of the detected issue.
9. Maintenance Scheduling: Predictive maintenance systems also help optimize maintenance schedules. Instead of performing maintenance at fixed intervals or after breakdowns, maintenance tasks can be scheduled based on the AI’s predictions, maximizing the uptime of equipment while minimizing unnecessary maintenance.
10. Continuous Learning: The AI models can be designed to learn from new data continuously. As more data is collected and analyzed, the models can adapt and improve their predictive capabilities over time.
By implementing AI-enabled predictive maintenance, industries can minimize downtime, reduce maintenance costs, and enhance overall operational efficiency. This proactive approach to maintenance helps prevent unexpected failures, ensuring smooth and reliable production processes.
#34 AI-powered facial recognition enhances security measures in various applications
AI-powered facial recognition enhances security measures in various applications through its ability to accurately and efficiently identify individuals based on their facial features. Here are some ways in which it achieves this:
1. **Access Control and Authentication**: In places like offices, airports, and secure facilities, AI-powered facial recognition systems can be used for access control. Instead of using traditional methods like key cards or PINs, individuals can simply have their faces scanned for identification. This enhances security by reducing the risk of unauthorized access due to lost or stolen cards or compromised passwords.
2. **Surveillance and Monitoring**: AI-powered facial recognition can be integrated with security cameras to monitor public spaces or private premises. It can automatically identify known individuals on watchlists and alert security personnel in real-time when a person of interest is detected, enabling a rapid response to potential threats.
3. **Law Enforcement and Investigations**: Facial recognition technology can be used by law enforcement agencies to match images of suspects captured on CCTV footage or public cameras with their existing databases of known criminals. This can help in identifying and locating suspects more efficiently.
4. **Border Security and Immigration Control**: At border checkpoints, airports, and immigration points, AI-powered facial recognition can be used to verify travelers’ identities against their passport or visa photos. This process can expedite the entry and exit of legitimate travelers while flagging potential security risks.
5. **Transaction Security**: In the financial sector, facial recognition can be used as an additional layer of security for verifying users during online transactions or mobile banking activities. This helps prevent unauthorized access to personal accounts and sensitive financial information.
6. **Attendance Tracking**: In educational institutions or workplaces, facial recognition systems can track attendance accurately without the need for manual recording, reducing the chances of fraudulent attendance.
7. **Lost and Found**: AI-powered facial recognition can also be used to help locate missing persons by comparing images of individuals in public places with databases of missing individuals.
8. **Event Security**: At large events or gatherings, facial recognition can help identify potential threats or individuals with restricted access to maintain a safe environment.
It’s important to note that while AI-powered facial recognition offers several benefits in enhancing security measures, there are also concerns related to privacy, bias, and misuse. Ethical considerations and robust regulations are necessary to ensure that the technology is deployed responsibly and with respect for individuals’ rights and privacy.
#35 AI-driven sentiment analysis helps businesses gauge customer feedback and satisfaction
AI-driven sentiment analysis helps businesses gauge customer feedback and satisfaction by using natural language processing (NLP) algorithms to automatically determine the emotional tone or sentiment behind written or spoken text. This technology enables businesses to understand how customers feel about their products, services, or brand, allowing them to make data-driven decisions and improve customer experiences. Here’s how it works:
1. Data collection: Businesses gather customer feedback from various sources, such as social media, customer surveys, online reviews, emails, and customer support interactions. These sources provide a vast amount of unstructured textual data.
2. Text preprocessing: Before analysis can take place, the raw text data needs to be cleaned and preprocessed. This involves tasks like removing irrelevant information, converting text to lowercase, removing punctuation, and handling special characters.
3. Sentiment analysis: AI models, often based on deep learning techniques like recurrent neural networks (RNNs) or transformers, are trained to understand the sentiment behind the text. During training, the model learns to associate certain patterns and words with positive, negative, or neutral sentiment.
4. Sentiment classification: Once the AI model is trained, it can classify new, unseen text into different sentiment categories, such as positive, negative, or neutral. Some more advanced models may even provide a continuous sentiment score, allowing a more nuanced understanding of customer feelings.
5. Data analysis and insights: The sentiment analysis results are aggregated and analyzed to gain insights into customer sentiment and satisfaction levels. Businesses can identify trends, common pain points, and areas of improvement. This information is valuable for making data-driven decisions to enhance products, services, and customer support.
6. Real-time monitoring: Sentiment analysis can be performed in real-time, enabling businesses to monitor customer sentiment as it changes over time. This is especially important during product launches, marketing campaigns, or during times of crisis, where quick action is required.
Benefits of AI-driven sentiment analysis for businesses:
1. Customer feedback analysis: It helps businesses process vast amounts of customer feedback quickly and efficiently, which would be challenging to do manually.
2. Improving customer experience: By understanding customer sentiment, businesses can identify pain points and areas of improvement to enhance their products and services.
3. Brand reputation management: Monitoring sentiment allows businesses to address negative feedback promptly and maintain a positive brand image.
4. Marketing and advertising optimization: Businesses can tailor their marketing strategies based on customer sentiment to resonate better with their target audience.
5. Competitive analysis: Sentiment analysis can also be used to compare customer sentiment between different companies in the same industry.
Overall, AI-driven sentiment analysis provides businesses with valuable insights into customer feelings, enabling them to respond effectively to customer needs and preferences and ultimately improve customer satisfaction and loyalty.
#36 AI enhances data analysis and insights, aiding decision-making processes across industries
AI enhances data analysis and insights by leveraging its capabilities in processing vast amounts of data, identifying patterns, and making predictions. Here’s how AI aids decision-making processes across industries:
1. Data Processing and Automation: AI can handle large volumes of data efficiently and automate repetitive tasks involved in data analysis. This streamlines the process, allowing organizations to quickly extract relevant information and focus on making informed decisions.
2. Pattern Recognition: AI algorithms excel at recognizing complex patterns and trends within data that might not be easily identifiable by humans. This ability enables businesses to spot correlations, outliers, and hidden insights that can impact decision-making positively.
3. Predictive Analytics: Through machine learning, AI can develop predictive models based on historical data, allowing businesses to forecast future trends and outcomes. This capability is valuable for demand forecasting, financial predictions, risk assessment, and more.
4. Personalization: AI-powered data analysis helps create personalized experiences for customers, tailoring products, services, and recommendations based on individual preferences and behaviors. This personalization improves customer satisfaction and loyalty.
5. Real-time Insights: AI can analyze data in real-time, providing up-to-date insights and alerts. This rapid analysis enables organizations to respond quickly to changing market conditions and customer demands, leading to better decisions.
6. Natural Language Processing (NLP): AI-driven NLP allows businesses to analyze unstructured data, such as customer feedback, social media posts, and online reviews, to gain valuable insights into customer sentiment and preferences.
7. Fraud Detection: In industries like finance and e-commerce, AI plays a crucial role in fraud detection by identifying suspicious patterns and behaviors, minimizing losses, and ensuring security.
8. Supply Chain Optimization: AI can optimize supply chains by analyzing data on inventory levels, production capacities, transportation routes, and demand patterns. This optimization leads to cost savings and improved efficiency.
9. Healthcare Decision Support: AI aids healthcare professionals in diagnosing diseases, predicting patient outcomes, and recommending treatment plans based on patient data, medical records, and research studies.
10. Risk Management: AI helps in assessing and managing risks across various sectors, including finance, insurance, and cybersecurity. It can identify potential risks and recommend mitigation strategies, leading to more secure and stable operations.
11. Resource Allocation: By analyzing data, AI can optimize resource allocation in fields like energy, agriculture, and manufacturing. This ensures resources are used efficiently, reducing waste and improving overall productivity.
In summary, AI enhances data analysis and insights by leveraging its computational power, pattern recognition abilities, and predictive capabilities to support decision-making processes across industries. By making sense of vast and complex datasets, AI enables organizations to make informed, data-driven choices, leading to improved efficiency, innovation, and competitive advantage.
#37 . AI-driven navigation apps provide real-time traffic updates and optimal routes for commuting
AI-driven navigation apps provide real-time traffic updates and optimal routes for commuting through a combination of various technologies and data sources. Here’s how they work:
1. GPS and Location Data: The foundation of navigation apps is the use of Global Positioning System (GPS) technology to determine the user’s current location. GPS satellites transmit signals, and the navigation app’s receiver calculates the user’s position based on the time it takes for these signals to reach the device.
2. Real-Time Traffic Data: Navigation apps collect real-time traffic data from various sources, such as government traffic monitoring systems, connected vehicles, and other users of the app. This information is constantly updated and provides insights into road conditions, traffic congestion, accidents, and road closures.
3. Machine Learning Algorithms: AI-driven navigation apps employ machine learning algorithms to analyze the vast amount of data they receive. These algorithms can recognize patterns and predict traffic conditions based on historical data. By continuously learning from user behavior and traffic patterns, they can make more accurate predictions over time.
4. Optimization Algorithms: To find the optimal route for a user’s destination, navigation apps use optimization algorithms that consider various factors, such as current traffic conditions, road closures, speed limits, and historical data. The goal is to minimize travel time and avoid congested areas, accidents, or roadblocks.
5. Crowdsourcing and User Feedback: Some navigation apps allow users to provide feedback on road conditions, traffic jams, or accidents they encounter during their commute. This crowdsourced data can be valuable for updating the real-time traffic information and improving the accuracy of the navigation app’s predictions.
6. Integration with Other Services: Navigation apps often integrate with other services, such as weather forecasts, public transportation data, and points of interest. This integration allows users to make informed decisions about their routes, considering not only traffic conditions but also weather, alternate transportation options, and nearby attractions.
7. Offline Maps: Many navigation apps offer the ability to download maps for offline use. This feature is especially useful in areas with poor or no network coverage, ensuring users can still access basic navigation functionality.
8. Continuous Improvement: As more users engage with the navigation app and provide feedback, the AI algorithms continuously learn and improve, resulting in more accurate traffic predictions and route recommendations over time.
Overall, AI-driven navigation apps leverage the power of real-time data, machine learning, optimization, and crowdsourcing to provide users with the most efficient and up-to-date routes for their daily commutes.
#38 AI-powered personal assistants schedule appointments, manage calendars, and send reminders
AI-powered personal assistants, like the one you’re interacting with right now, use a combination of natural language processing (NLP), machine learning, and integrated APIs to schedule appointments, manage calendars, and send reminders. Here’s a general overview of how these tasks are accomplished:
1. **Natural Language Processing (NLP)**: NLP is a key component of AI-powered personal assistants. It enables the assistant to understand and interpret human language, both written and spoken. When you interact with the assistant, it processes your input, extracts relevant information, and determines the intent of your request.
2. **Intent Recognition**: After understanding your input, the assistant identifies the intent behind your request. For example, if you say, “Schedule a meeting with John tomorrow at 3 PM,” the intent is to schedule an appointment.
3. **Calendar Integration**: The AI assistant is connected to your calendar application, such as Google Calendar or Microsoft Outlook. It can access your calendar, view existing appointments, and find suitable time slots for new events.
4. **Machine Learning Algorithms**: AI-powered personal assistants utilize machine learning algorithms to analyze past interactions and learn from user behavior. This learning process allows the assistant to personalize responses and become more efficient over time.
5. **Appointment Scheduling**: Using the information obtained from your request and the insights from your calendar, the assistant proposes potential time slots for the appointment. It considers factors like availability, preferences, and conflicting events to suggest the most suitable options.
6. **Confirmation and Reminders**: Once a suitable time slot is selected, the assistant sends you a confirmation message. Additionally, it can set up reminders for the upcoming appointment to ensure you don’t miss it. Reminders can be sent via email, push notifications, or other preferred communication channels.
7. **Natural Language Generation (NLG)**: After finalizing the details of the appointment, the assistant generates natural language responses to communicate with you in a human-like manner. This NLG component ensures that the messages you receive are coherent and easily understandable.
8. **API Integrations**: Personal assistants often integrate with various third-party services through APIs (Application Programming Interfaces). For example, they may connect with location services, contact databases, or video conferencing platforms to streamline the appointment-setting process.
9. **Security and Privacy**: To handle sensitive information like calendars and appointments, AI-powered personal assistants prioritize security and privacy. They use encryption and follow industry-standard protocols to safeguard your data.
By combining these technologies and capabilities, AI-powered personal assistants can efficiently manage appointments, calendars, and reminders, making them valuable tools for individuals and professionals alike.
#39 AI facilitates speech synthesis, making it possible to generate lifelike computer-generated voices
AI facilitates speech synthesis by leveraging deep learning techniques, particularly in the field of Natural Language Processing (NLP) and specifically using models like WaveNet and Tacotron. Here’s a simplified explanation of how AI enables lifelike computer-generated voices:
1. **Data Collection and Preprocessing:** To train a lifelike speech synthesis model, a large dataset of audio recordings is collected. These recordings typically consist of real human voices reading a diverse set of sentences or phrases. The data is then preprocessed to extract useful features and prepare it for training.
2. **Deep Learning Models:** Two key types of deep learning models are commonly used in speech synthesis:
– **Text-to-Speech (TTS) Models:** These models convert written text into spectrograms, which are visual representations of the audio frequency content over time. TTS models use recurrent neural networks (RNNs), long short-term memory networks (LSTMs), or transformer-based architectures to map text input to spectrogram output.
– **Waveform Generation Models:** These models take the spectrogram output from TTS models and generate raw waveforms that correspond to the audio. They employ techniques like WaveNet, WaveGlow, or similar neural network architectures to produce high-fidelity audio waveforms.
3. **Training:** The TTS and waveform generation models are trained on the preprocessed dataset. During training, the models learn to map textual inputs to corresponding high-quality speech waveforms.
4. **Transfer Learning and Fine-tuning:** To achieve lifelike voices, some speech synthesis models use transfer learning. A pre-trained model on a large corpus of general language data is fine-tuned on a smaller dataset containing specific voice characteristics and speaking style. This fine-tuning process helps capture individual nuances, accents, and expressive qualities of the desired voice.
5. **Prosody and Intonation:** To make the generated speech sound more natural, AI models pay attention to prosody and intonation. Prosody refers to the rhythm, stress, and intonation patterns in speech that convey meaning and emotion. By incorporating prosody into the model, the generated speech becomes more expressive and lifelike.
6. **Post-processing and Voice Quality:** After the initial speech synthesis, some additional post-processing steps may be applied to enhance voice quality further. This could involve smoothing out generated waveforms, suppressing background noise, or adjusting pitch and tone.
7. **Real-time Synthesis:** Advanced AI models can achieve real-time speech synthesis, allowing for dynamic and interactive applications that generate speech on-the-fly based on user inputs.
By combining these deep learning techniques, AI systems can create lifelike computer-generated voices that are nearly indistinguishable from human speech. This has revolutionized various applications, such as virtual assistants, audiobook narration, voice-overs, accessibility tools for visually impaired individuals, and more.
#40 AI-powered personal finance apps offer budgeting and expense tracking features
AI-powered personal finance apps offer budgeting and expense tracking features through a combination of advanced algorithms, data analysis, and machine learning techniques. Here’s how they typically work:
1. Data Aggregation: Users link their bank accounts, credit cards, and other financial accounts to the app. The app securely collects and aggregates transaction data from these accounts, allowing users to view all their financial information in one place.
2. Categorization: The AI algorithms in the app analyze the transactions and use natural language processing (NLP) techniques to categorize them into different expense categories, such as groceries, utilities, entertainment, etc. Initially, this categorization might be based on pre-defined rules, but as the AI learns from the user’s spending patterns, it can improve its accuracy.
3. Spending Analysis: The AI examines the user’s historical spending patterns to understand their habits, identify trends, and detect any irregularities or unexpected expenses.
4. Budgeting: Based on the user’s income, expenses, and financial goals, the AI helps create a personalized budget. It suggests budgeting strategies and allocates specific amounts for different expense categories to help users stay on track.
5. Expense Tracking: Users can input cash transactions or expenses from unsupported accounts manually, and the AI integrates this information into their overall financial picture.
6. Real-time Insights: The AI provides real-time updates on spending, remaining budget, and progress towards financial goals. Users can access this information through the app’s dashboard or receive notifications/alerts to stay informed.
7. Predictive Analysis: The AI uses historical data and user behavior patterns to make predictions about future expenses. It can forecast potential overspending or alert users about upcoming bills and financial commitments.
8. Smart Recommendations: AI-powered apps can offer personalized recommendations to optimize spending, save money, or invest better. For instance, they might suggest switching to a different credit card with better rewards, recommend budget adjustments, or propose investment options based on the user’s risk tolerance and financial goals.
9. Security and Privacy: Ensuring the security and privacy of users’ financial data is paramount. AI-powered apps use encryption and follow stringent security protocols to protect sensitive information.
10. Continuous Learning: The AI models continuously learn and improve from user interactions and feedback, becoming more accurate and tailored to each individual’s financial situation over time.
By combining these AI-driven features, personal finance apps empower users with a comprehensive understanding of their financial health, help them manage their money more effectively, and work towards achieving their financial goals.
#41 AI-driven e-commerce platforms suggest products based on user preferences and buying history
AI-driven e-commerce platforms suggest products to users based on a combination of user preferences, buying history, and various AI techniques. Here’s a general overview of how it works:
1. **Data Collection and User Profiling:** E-commerce platforms collect vast amounts of data from users, including their browsing behavior, search queries, product views, purchases, and interactions with the website or app. This data is used to build user profiles, which contain information about the individual’s preferences, interests, and buying habits.
2. **Collaborative Filtering:** One of the common AI techniques used in recommendation systems is collaborative filtering. It analyzes the behavior of multiple users and identifies patterns of product preferences among users with similar tastes. When a user shows interest in a product or makes a purchase, the system looks for other users who have shown similar behavior and suggests products that those similar users have also liked or bought.
3. **Content-Based Filtering:** Content-based filtering relies on the attributes of the products themselves. Each product is associated with a set of descriptive features, such as category, brand, price, size, color, etc. The system suggests products that are similar in attributes to the ones a user has previously shown interest in or purchased.
4. **Machine Learning Algorithms:** Advanced machine learning algorithms, such as decision trees, neural networks, and matrix factorization, are used to process the data and make predictions about user preferences. These algorithms continuously learn from new data to improve the accuracy of their recommendations over time.
5. **Natural Language Processing (NLP):** NLP allows the platform to understand the context of user queries and product descriptions better. This way, the system can suggest products more accurately based on user search queries or provide more relevant product descriptions.
6. **Real-Time Personalization:** As users interact with the platform, their preferences may change. AI-driven e-commerce platforms continuously update user profiles and adjust their recommendations in real-time to reflect these changes.
7. **A/B Testing:** To enhance the effectiveness of their recommendation systems, e-commerce platforms often perform A/B testing. This involves testing different recommendation algorithms or strategies on a subset of users to determine which ones yield the best results in terms of click-through rates, conversions, and user satisfaction.
8. **Feedback Loop:** The platform also relies on user feedback to refine its recommendations. When users provide explicit feedback (e.g., ratings or reviews) on the suggested products, the system can use this information to further fine-tune the recommendations and ensure they align more closely with user preferences.
By combining these techniques and constantly updating their models with new data, AI-driven e-commerce platforms aim to provide personalized and relevant product recommendations that encourage users to make more purchases and enhance their overall shopping experience.
#42 AI assists in wildlife conservation by analyzing data and identifying patterns in animal behaviors
AI plays a crucial role in wildlife conservation by analyzing vast amounts of data and identifying patterns in animal behaviors in various ways:
1. **Image and Video Analysis**: AI algorithms can analyze images and videos captured by cameras in the wild, such as camera traps or drones. By using computer vision techniques, AI can automatically identify and classify different species, track their movements, and even estimate population sizes.
2. **Acoustic Monitoring**: AI can process audio recordings from remote sensors to identify specific animal sounds or vocalizations. For example, it can distinguish between different bird calls or identify the sounds of endangered species, helping researchers monitor their presence and behavior.
3. **GPS Tracking Data**: Many animals in the wild are fitted with GPS tracking collars or tags. AI can analyze this tracking data to understand animal movement patterns, migration routes, and habitat preferences, which can provide insights into their behavior and ecological needs.
4. **Satellite Imagery**: Remote sensing data from satellites can be used to monitor large areas and track changes in ecosystems. AI algorithms can analyze this data to detect deforestation, changes in vegetation, and other environmental indicators that can affect wildlife behavior and habitat.
5. **Machine Learning Models**: AI employs machine learning models to process and interpret data. These models can recognize patterns and correlations in large datasets, helping scientists understand how factors such as weather, food availability, or human activities impact animal behavior.
6. **Predictive Analytics**: By analyzing historical data, AI can make predictions about future animal behaviors or potential threats. For example, it can forecast animal migration patterns or identify areas where poaching is more likely to occur.
7. **Real-time Alerts**: AI-powered systems can provide real-time alerts to conservationists when certain behaviors are detected. For instance, they can notify researchers about the presence of a rare or endangered species in a particular area, prompting swift action to protect it.
8. **Behavioral Analysis**: AI can assist in identifying abnormal behaviors in wildlife, which may indicate disease or stress. Early detection of such issues can help prevent potential outbreaks or mitigate threats to the animal population.
9. **Conservation Planning**: AI can assist in developing effective conservation strategies by analyzing data from multiple sources and identifying critical areas for wildlife protection. It can also optimize the placement of conservation efforts to have the most significant impact.
Overall, AI’s ability to process vast amounts of data and recognize patterns quickly and accurately makes it a valuable tool in wildlife conservation, enabling researchers and conservationists to make informed decisions and take proactive measures to protect and conserve endangered species and their habitats.
#43 AI-powered language translation devices enable real-time communication in foreign countries
AI-powered language translation devices enable real-time communication in foreign countries through a combination of advanced technologies and machine learning algorithms. These devices leverage natural language processing (NLP) techniques, speech recognition, and machine translation to facilitate seamless communication between people who speak different languages. Here’s how they work:
1. Speech Recognition: The AI-powered translation device has a built-in microphone that captures the spoken language. The device converts the speech input into a digital format through speech recognition algorithms. These algorithms analyze the sound patterns and phonetics to identify the words being spoken.
2. Natural Language Processing (NLP): Once the speech is converted into text, the device uses NLP algorithms to understand the meaning and context of the words. NLP enables the device to interpret the sentence structure, grammar, and semantics of the language.
3. Machine Translation: After understanding the input text, the AI translation device employs machine translation algorithms to convert the text into the desired target language. Machine translation involves using vast datasets of multilingual content to learn the patterns and correlations between languages.
4. Language Generation: Once the translation is complete, the device utilizes natural language generation (NLG) algorithms to convert the translated text into spoken language. This step allows the device to verbalize the translated message in a human-like voice.
5. Real-Time Output: All of the above steps occur in real-time, enabling instant translation of spoken words. The AI-powered translation device provides the translated output immediately, allowing the users to engage in a conversation without significant delays.
6. Continuous Learning: Many AI-powered language translation devices are also equipped with online connectivity, allowing them to access the internet and cloud-based language databases. This feature enables the devices to continuously improve their translation accuracy by learning from new data and user interactions.
Thanks to these advanced technologies and algorithms, AI-powered language translation devices have revolutionized the way people communicate across language barriers. They have become essential tools for travelers, business professionals, and individuals who regularly interact with people from different linguistic backgrounds, enabling them to communicate effectively and seamlessly in real-time.
#44 AI improves energy efficiency in smart buildings by optimizing heating, cooling, and lighting systems
AI can significantly improve energy efficiency in smart buildings by optimizing heating, cooling, and lighting systems through the following mechanisms:
1. Data Analytics and Pattern Recognition: AI algorithms can analyze vast amounts of data collected from various sensors installed in the building. This data includes information about temperature, humidity, occupancy, and external weather conditions. By recognizing patterns and trends in the data, AI can make informed decisions on how to adjust the building’s systems for optimal energy usage.
2. Predictive Modeling: AI can use historical data and machine learning techniques to build predictive models. These models can anticipate future energy needs based on factors like occupancy patterns, weather forecasts, and historical energy consumption. By predicting energy demand, AI can pre-adjust heating, cooling, and lighting systems to be more energy-efficient.
3. Adaptive Control and Automation: AI can dynamically adjust heating, cooling, and lighting settings in real-time based on current conditions and occupancy. For instance, AI can automatically lower heating or cooling when a room is unoccupied or adjust lighting levels based on natural light availability. Adaptive control ensures that energy is used only when and where it is needed, reducing wastage.
4. Demand Response Management: AI can participate in demand response programs where it monitors the grid’s energy demand and supply conditions. During peak demand periods, AI can optimize the building’s energy consumption by temporarily reducing heating, cooling, or lighting, helping to alleviate stress on the power grid.
5. Optimal Scheduling: AI can create optimal schedules for heating, cooling, and lighting systems based on energy tariffs, peak and off-peak periods, and user preferences. This ensures that energy-intensive operations are performed during periods of lower energy costs, maximizing energy efficiency.
6. User Behavior Analysis: AI can learn from occupants’ behaviors and preferences over time. By understanding how users interact with the building environment, AI can adjust systems to align with their preferences while still maintaining energy efficiency.
7. Fault Detection and Maintenance: AI can identify inefficiencies and faults in the building’s energy systems and equipment. By quickly detecting and alerting maintenance staff to these issues, AI helps ensure that systems are operating at their highest efficiency levels.
8. Continuous Learning and Improvement: AI algorithms can adapt and improve their performance over time through machine learning. As more data is collected and analyzed, AI can refine its optimization strategies to achieve even greater energy efficiency.
By incorporating AI-driven optimization techniques into smart buildings, energy consumption can be significantly reduced, leading to cost savings, reduced environmental impact, and enhanced comfort for building occupants.
#45 AI enhances content creation, generating automated summaries and article suggestions
AI can enhance content creation by leveraging natural language processing (NLP) and machine learning algorithms. Here’s how it can generate automated summaries and article suggestions:
1. **Automated Summaries:**
AI-powered summarization systems can analyze large bodies of text and generate concise summaries that capture the main points and key information. There are two main approaches to automated summarization:
– **Extractive Summarization:** In this method, the AI system identifies and extracts the most important sentences or phrases directly from the original text. It ranks sentences based on relevance, coherence, and importance and selects the top sentences to form the summary.
– **Abstractive Summarization:** This approach involves generating summaries by paraphrasing and rephrasing content from the original text. The AI system understands the context and meaning of the input text and creates new sentences that convey the main ideas in a more condensed form.
2. **Article Suggestions:**
AI can assist content creators by suggesting topics and ideas for articles based on various sources of information:
– **Keyword and Trend Analysis:** AI can analyze popular keywords and trends in specific domains to identify relevant and timely topics for content creation. It can also analyze search engine data and social media trends to suggest topics that are currently popular.
– **User Behavior Analysis:** AI can analyze user behavior on websites, social media platforms, and content sharing sites to understand what kind of content users are engaging with the most. It can then suggest similar topics that are likely to resonate with the target audience.
– **Content Gap Analysis:** By analyzing existing content in a particular niche or domain, AI can identify content gaps or areas that are not well-covered. It can suggest topics that fill these gaps and provide valuable information to readers.
3. **Content Enhancement:**
AI can also help content creators improve their writing by providing real-time suggestions and feedback:
– **Grammar and Spelling Checking:** AI-powered language models can check for grammar errors, spelling mistakes, and punctuation issues to ensure that the content is error-free.
– **Writing Style and Tone Suggestions:** AI can suggest improvements to the writing style and tone, depending on the target audience and purpose of the content. For example, it can help adapt content for a more formal or casual tone.
– **Enhancing Clarity and Coherence:** AI can provide suggestions to improve the clarity and coherence of the content, ensuring that the ideas are well-organized and easy to understand.
Overall, AI’s ability to process and understand large volumes of text data enables it to enhance content creation by generating summaries, suggesting relevant topics, and providing valuable feedback to writers, making the content creation process more efficient and effective.
#46 AI-driven chatbots provide mental health support and resources to users in need
AI-driven chatbots can provide mental health support and resources to users in need through a combination of natural language processing, machine learning, and access to a vast database of mental health-related information. Here’s how they can achieve this:
1. **Active Listening and Emotional Support:** Chatbots are designed to actively listen to users’ concerns and emotions without judgment. They can use natural language processing to understand the user’s messages and respond in a compassionate and empathetic manner, making users feel heard and supported.
2. **Assessment and Screening:** AI chatbots can use evidence-based questionnaires and screening tools to assess a user’s mental health condition. By asking specific questions about symptoms and experiences, the chatbot can provide initial insights into potential mental health issues.
3. **Immediate Responses:** AI chatbots can offer immediate responses and support 24/7, which is especially beneficial for individuals who may be in distress outside of regular business hours or in regions with limited access to mental health services.
4. **Providing Information and Resources:** These chatbots can offer information about various mental health conditions, treatment options, coping strategies, and self-help resources. They can also provide links to reputable websites and articles for further reading.
5. **Personalized Recommendations:** Through machine learning algorithms, chatbots can gather and analyze data from users’ interactions, providing personalized recommendations based on individual needs and preferences.
6. **Crisis Intervention:** AI-driven chatbots can be programmed to identify signs of a mental health crisis, such as suicidal ideation, and offer immediate intervention by connecting users to emergency hotlines or suggesting emergency contacts.
7. **Referral to Professionals:** If the chatbot recognizes that a user’s condition requires professional help beyond what it can provide, it can suggest seeking support from licensed mental health professionals or direct the user to nearby mental health services.
8. **Continuity of Care:** Chatbots can offer ongoing support and follow-up interactions, checking in with users regularly to track their progress, provide encouragement, and reinforce positive behaviors.
9. **Anonymous and Non-Judgmental Environment:** Some individuals may find it easier to open up about their mental health struggles to an AI chatbot due to the anonymity it offers. The lack of judgment allows users to express themselves more freely.
10. **Language Accessibility:** AI chatbots can communicate in multiple languages, making mental health support accessible to a more diverse range of users around the world.
However, it’s important to note that AI chatbots are not a substitute for professional mental health care. They can complement existing mental health services, offer immediate support, and disseminate helpful information, but they cannot replace the expertise and personalized care provided by qualified mental health professionals. If someone is experiencing severe mental health issues or emergencies, it’s crucial to seek help from a licensed mental health practitioner or a crisis hotline.
#47 AI facilitates medical diagnoses through analyzing symptoms and medical history
AI facilitates medical diagnoses through a process known as medical decision support systems (MDSS) or clinical decision support systems (CDSS). These systems leverage the power of artificial intelligence and machine learning algorithms to assist healthcare professionals in diagnosing medical conditions based on symptoms and medical history. Here’s how they work:
1. Data Collection: The first step involves gathering relevant patient data, including symptoms, medical history, test results, imaging studies, and other clinical information. This data is typically stored in electronic health records (EHRs) or other healthcare databases.
2. Data Preprocessing: AI systems require clean and structured data to function effectively. Preprocessing involves organizing and standardizing the data to ensure it is ready for analysis.
3. Feature Extraction: Features are specific data points or attributes that are relevant to the diagnosis. AI algorithms analyze and extract important features from the preprocessed data, focusing on factors that may be indicative of certain medical conditions.
4. Machine Learning Algorithms: AI uses various machine learning techniques, such as supervised learning, unsupervised learning, and deep learning, to train models on historical patient data. The training process helps the AI system learn patterns, associations, and correlations between symptoms, medical history, and specific medical conditions.
5. Diagnosis and Prediction: Once the AI model is trained, it can be used to predict or diagnose medical conditions in new patients. When a patient’s symptoms and medical history are input into the system, the AI analyzes the data and provides potential diagnoses or probabilities of different conditions.
6. Decision Support: AI-powered medical diagnosis systems don’t replace healthcare professionals; instead, they offer valuable decision support. The system presents possible diagnoses along with the level of confidence for each one. Healthcare professionals can then use this information to make more informed decisions about patient care.
7. Continuous Learning: AI systems can continuously learn and improve over time as they receive more data and feedback from healthcare professionals. This iterative process enhances their accuracy and performance in diagnosing medical conditions.
Benefits of AI in Medical Diagnoses:
– Speed: AI can process vast amounts of data quickly, leading to faster diagnosis and treatment planning.
– Accuracy: AI can identify subtle patterns and associations in data that might be challenging for human experts to notice, enhancing diagnostic accuracy.
– Standardization: AI can ensure a consistent approach to diagnoses by following evidence-based guidelines and best practices.
– Handling Complexity: In complex cases with numerous symptoms and factors, AI can efficiently analyze and weigh various parameters for a more comprehensive diagnosis.
However, it’s important to note that while AI can be a valuable tool in medical diagnoses, it should never replace the expertise and judgment of trained healthcare professionals. AI systems are meant to complement and assist medical professionals in their decision-making processes, not replace them.
#48 AI improves voice-controlled smart home devices, making them more accurate and responsive
AI can significantly enhance the performance of voice-controlled smart home devices, making them more accurate and responsive through various techniques and advancements. Here are some ways AI achieves this:
1. Natural Language Processing (NLP): AI-powered NLP algorithms enable smart home devices to better understand and interpret spoken language. NLP algorithms use techniques like speech recognition, language modeling, and syntactic parsing to accurately comprehend user commands and queries.
2. Machine Learning Models: AI employs machine learning models, especially deep learning-based approaches, to continuously improve voice recognition accuracy over time. These models are trained on vast datasets containing diverse speech patterns, accents, and languages, enabling the devices to adapt and become more accurate with each interaction.
3. Contextual Understanding: Advanced AI algorithms enable smart home devices to understand context and intent. By analyzing the context of a conversation, devices can respond more accurately to ambiguous or complex queries. For instance, a command like “Turn off the lights” is better understood when the device knows which room the user is in or which lights are currently on.
4. Data-driven Personalization: AI algorithms leverage user data to personalize the device’s responses and improve accuracy. As the device learns more about the user’s preferences, habits, and speech patterns, it can fine-tune its responses to suit individual needs.
5. Real-time Feedback and Adaptation: AI-powered devices can receive real-time feedback from users, allowing them to continuously improve their accuracy. When users correct misinterpreted commands, the device can use this information to adjust its recognition models and avoid similar mistakes in the future.
6. Noise Reduction and Signal Processing: AI can filter out background noise and improve speech signal processing, making it easier for smart home devices to accurately capture voice commands even in noisy environments.
7. Cloud-Based Processing: Many voice-controlled smart home devices use cloud-based AI processing, allowing them to leverage vast computational resources to process complex voice commands rapidly. This offloading of processing to the cloud can lead to quicker and more accurate responses.
8. Active Learning: Some AI systems use active learning techniques, where the device can proactively seek clarifications from the user when it encounters ambiguous commands. This helps improve accuracy and reduces errors over time.
9. Multi-modal Integration: AI can combine voice inputs with other sensor data (e.g., cameras, motion sensors) to better understand user intent. Multi-modal integration adds another layer of context, allowing for more accurate and responsive interactions.
Overall, AI plays a crucial role in the continuous improvement of voice-controlled smart home devices, helping them become more accurate, reliable, and responsive to user commands and preferences.
#49 AI-powered virtual tutors offer personalized learning experiences to students outside the classroom
AI-powered virtual tutors offer personalized learning experiences to students outside the classroom through the use of artificial intelligence algorithms and machine learning techniques. Here’s how they achieve this:
1. **Adaptive Learning:** Virtual tutors use AI algorithms to assess a student’s strengths and weaknesses by analyzing their performance, learning style, and progress. Based on this analysis, the tutor adapts the learning content and pace to match the individual student’s needs, ensuring they receive appropriate challenges and support.
2. **Individualized Content:** AI tutors can access vast repositories of educational content, including texts, videos, quizzes, and interactive exercises. They select and present the most relevant and appropriate content for each student, considering their learning preferences and comprehension levels.
3. **Natural Language Processing (NLP):** AI-powered tutors often use NLP to communicate with students in natural language. This allows students to ask questions and engage in conversations with the tutor, simulating a more human-like interaction and catering to different learning styles.
4. **Continuous Assessment:** Virtual tutors continuously monitor the student’s progress, evaluating their performance in real-time. Through data analysis, the tutor can identify areas where the student is struggling and provide additional support or practice exercises to reinforce learning.
5. **Feedback and Guidance:** AI tutors can give personalized feedback to students on their assignments, assessments, and practice exercises. This feedback is tailored to the individual’s mistakes and areas of improvement, helping them understand their errors and how to rectify them.
6. **24/7 Availability:** Virtual tutors are available at any time, allowing students to learn at their own pace and convenience. This accessibility ensures that students can receive support and guidance whenever they need it, which is especially beneficial for self-paced learners.
7. **Emotional Intelligence:** Some advanced AI tutors are equipped with emotional intelligence capabilities. They can recognize and respond to students’ emotions, providing encouragement, motivation, and empathy as needed, creating a supportive and positive learning environment.
8. **Personalized Study Plans:** AI-powered virtual tutors can create personalized study plans for each student based on their learning goals, academic strengths, and areas for improvement. These plans help students stay organized and focused on their learning objectives.
9. **Learning Analytics:** Virtual tutors use learning analytics to track and analyze students’ performance data. This data can be used to generate insights for educators, parents, or the students themselves, helping them understand their learning patterns and progress.
10. **Continuous Improvement:** AI tutors can also improve over time through machine learning. They can analyze their own interactions and effectiveness, using this data to enhance their teaching methods and become better at catering to individual student needs.
By leveraging these AI-driven features, virtual tutors provide a personalized and adaptive learning experience that complements traditional classroom education and supports students in their learning journey.
#50 AI is continually evolving
AI is indeed continually evolving, and its progress can be attributed to several key factors:
1. **Advancements in Research:** AI is driven by ongoing research in the field of machine learning and artificial intelligence. Researchers and scientists are constantly developing new algorithms, models, and techniques to improve AI’s capabilities, efficiency, and accuracy.
2. **Data Availability:** AI systems thrive on data, and the availability of large and diverse datasets has grown exponentially in recent years. More data allows AI models to learn and generalize better, leading to improved performance in various tasks.
3. **Computational Power:** The increasing computational power of modern hardware, such as GPUs and specialized AI accelerators, has enabled the training of larger and more complex AI models. This has significantly improved the performance and efficiency of AI algorithms.
4. **Deep Learning:** Deep learning, a subset of machine learning based on neural networks with multiple layers, has revolutionized AI in the past decade. Deep learning models have shown remarkable performance in tasks like image recognition, natural language processing, and more.
5. **Transfer Learning:** Transfer learning allows AI models to leverage knowledge gained from one task or domain and apply it to another. This technique has led to faster and more efficient learning, as models don’t need to start from scratch for every new task.
6. **Reinforcement Learning:** Reinforcement learning is a type of machine learning where an AI agent learns through trial and error, receiving feedback in the form of rewards or penalties. This approach has shown significant progress in areas like game-playing agents and robotics.
7. **AI Ethics and Regulations:** As AI becomes more widespread, there is a growing emphasis on AI ethics and responsible development. Clear guidelines and regulations promote the development of safe and beneficial AI systems, which ensures long-term sustainable growth.
8. **Industry Adoption:** AI is being adopted in various industries, including healthcare, finance, automotive, and more. Real-world applications drive further research and development, leading to practical and innovative use cases.
9. **Interdisciplinary Collaboration:** AI’s evolution is not confined to computer science alone. Collaboration with experts from diverse fields, such as neuroscience, psychology, linguistics, and robotics, brings fresh perspectives and accelerates progress.
10. **Open-Source Community:** The open-source AI community fosters collaboration and knowledge sharing. Many breakthroughs in AI have originated from open-source projects, making cutting-edge AI accessible to a wider audience.
11. **Natural Language Processing (NLP) Advancements:** Improvements in NLP have enabled AI systems to understand and generate human language more effectively. This has led to the development of more sophisticated chatbots, language translators, and virtual assistants.
12. **Edge Computing:** The shift towards edge computing, where AI processing is done on local devices instead of centralized servers, allows for faster and more efficient AI applications, especially in resource-constrained environments.
These factors, among others, contribute to the continuous evolution of AI and ensure that it remains at the forefront of technological progress, impacting various aspects of our daily lives and shaping the future of technology.
Conclusion
Artificial Intelligence has revolutionized the way we live, work, and interact with the world around us. From virtual assistants to autonomous vehicles, AI’s impact on everyday life is evident in various sectors. As AI technology continues to advance, its potential to improve efficiency, convenience, and overall quality of life becomes even more promising. However, it is essential to address the ethical considerations surrounding AI development and usage to ensure a responsible and inclusive future for this transformative technology.